Creating the ideal dataset: everything you need to know
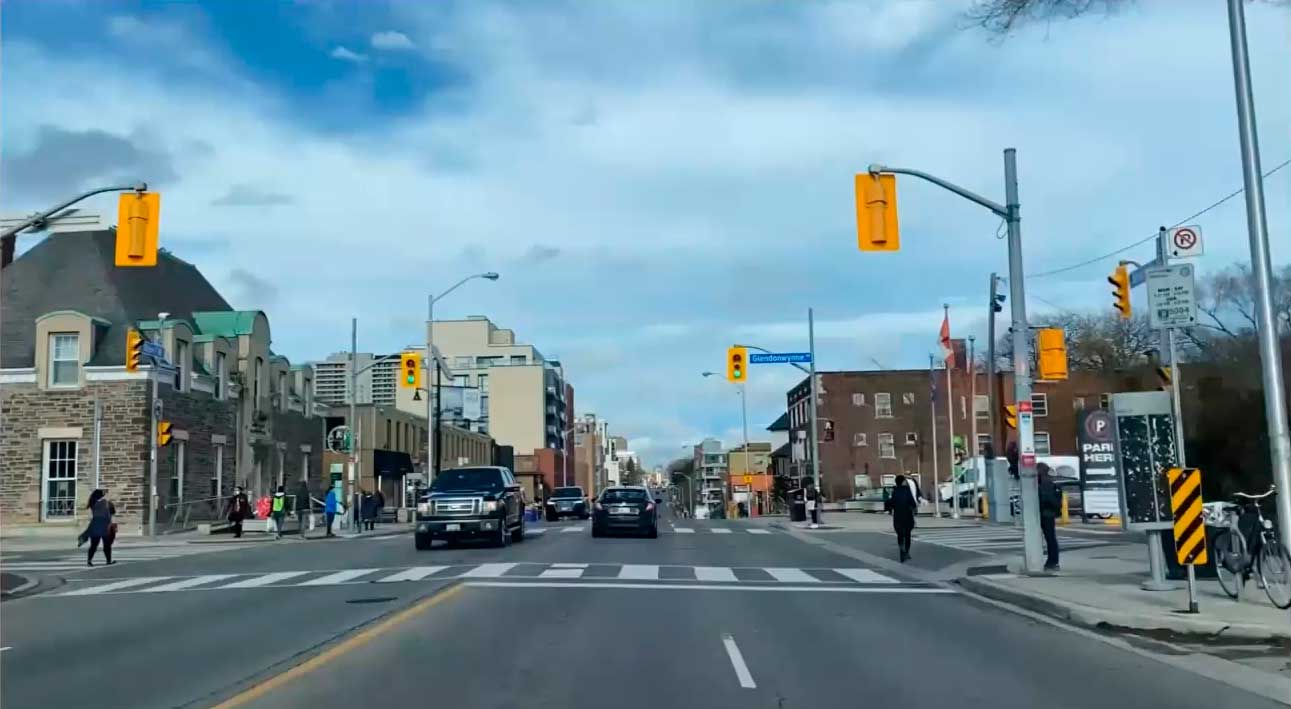
What makes an Ideal dataset? What are the pros and cons of open source datasets?
Joined webinar with our partner Cloudafactory will cover these questions and many more.
Here is what we will talk about in the webinar:
- What makes the ideal data set
- The pros and cons of open source, pre-labeled data set vs. building your own
- Best practices for building your own custom data labeled set
- Dealing with sensitive data in the right way (specifically medical imaging)
- What to do if the data doesn’t exist
The webinar content summary.
Creating dataset | Keymakr
What is ideal data set in image segmentation processing?
A good dataset should be:
- Diverse
- Represent the real life as much as possible.
- Have a high quality data. Here it gets interesting. What is “high quality”?
- If we are talking about image annotation projects - doesn’t necessary means high resolution or clear images- high quality data means that the images represent the real-life scenario. If the machine learning image processing should recognize people in the dark or vehicles driving in for, high quality images are exactly the opposite of what we want.
- Minimal bias. There is always a bias, we need to be aware of it and try to minimalize it. For example, Autonomous vehicles training data: using footage of year-around HW driving collected in California only, would be a classic bias as the output of this Computer vision model would not be relevant to New York. The weather is just not the same!
- Enriching the data: the quality of the annotations and the precision of the image detection is especially important.
- Searchable and organized. Should be easy to navigate through. Here the projects on image processing best practices come in play.
The pros and cons of open source, pre-labeled data set vs. building your own
There are multiple open datasets available online, and we can download a dataset for free or for a low price. But what is the cost of the “free”?
Pros of an open dataset:
Available immediately
Free
The cons:
- Generic and high level
- Not specified & not specific
- Not enough data
- Data your need does not exist or can be found anywhere
- Poor labeling quality
- Regions based data
Bottom line is, if you can find an open dataset that suits your needs, go for it. Most likely this will not be the case
- Best practices for building your own custom dataset:
Collect and annotate in tiers! For example, if you need to collect and annotate 100,000 images, don’t do all 100k in one go. Break it to small pieces, collect 1000, validate, see how your machine learning (ML) model responds, adjust your requirements then collect again.
Make sure to take in account the “what makes a good dataset” list!
- Dealing with sensitive data:
There are many aspects to that, starting form privacy issues to copyrights and ownership.
Some common practices is to make a pointer to where the data is without downloading it or anonymizing the data.
- What to do if the data doesn’t exist
If it doesn’t exist – create it! Quite simple. Some of the projects that we did for data creation:
Collect dashcam videos from various locations around the globe.
Set up a photo studio, and with people’s full consent take images and videos of people’s faces, eye IRIS, gaze, etc.
Collecting data from 1000’s of mobile phones: sensory information as well as taking images of objects/ places.
For each data creation project, we set up a whole production and create the data that needed for this specific project.
For more detailed information, please watch the full webinar.
Need data collection and data creation, let’s talk!
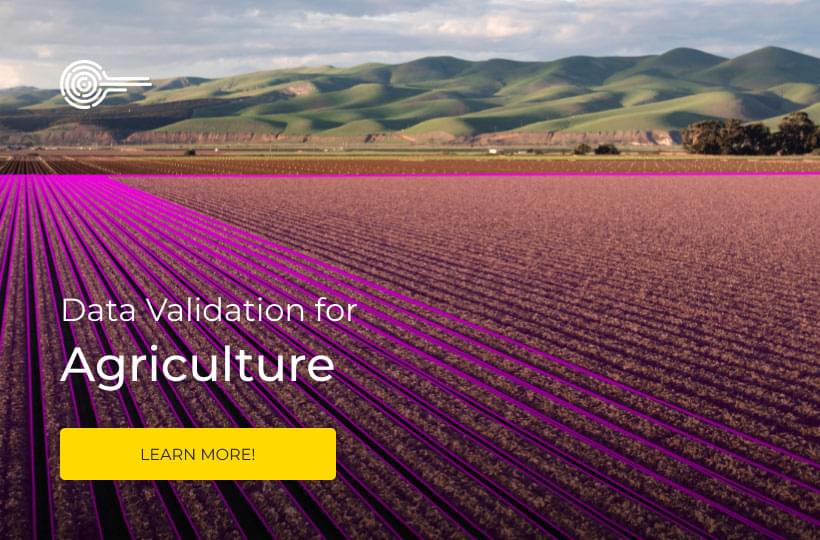