Combating Climate Change With Computer Vision Video Annotation
In the past few years, there has been increasingly more news about the harmful effects of climate change on our planet. Luckily, people are working on this issue daily. Scientists are studying the causes of climate change. And engineers are discovering ways to mitigate its effects using computer vision video annotation.
Climate change requires global solutions. Identifying the impacts of climate change is the first step toward change. But leaders need tools to help identify datasets patterns that might go unnoticed. And it turns out that computer vision and machine learning are doing that.
Applications for Computer Vision and Machine Learning in Fighting Climate Change
Computer vision is an artificial intelligence (AI) tool. It focuses on processing images and videos to understand patterns. For example, researchers can train an AI system using satellite images. For example, systems can identify coral reefs or mangrove forests. In addition, researchers can use computer vision to track environmental changes.
Machine learning is an approach that uses computers to solve problems based on data. It has many new applications and technologies to tackle climate change issues. For example, without human input, computers can learn from data. Also, they can identify objects in photos or predict weather conditions.
Monitoring Glaciers
One of the most exciting ways scientists use these tools is to monitor glaciers. The problem with studying glaciers is that they're far away and dangerous. It's difficult for researchers on foot to get close enough for detailed measurements.
Instead of approaching glaciers, scientists use computer vision video annotation to analyze satellite images. Then, they can identify ice volume and velocity changes over time. This information is helpful for modeling purposes. It helps understand how glaciers respond to conditions (like warming temperatures).
Measuring Carbon Dioxide Levels
Computer vision can help track environmental data. For example, how much carbon dioxide enters the atmosphere or how much rainfall an area gets. These tools collect vast amounts of data daily but can only do so much. So systems need people to tag those images with metadata to know what they're seeing.
Machines can identify land cover, including forests, grasslands, and deserts. This information can help estimate the carbon dioxide in the atmosphere. It can also determine how much CO2 humans release through burning fossil fuels.
Detecting Changes in the Weather and Environment
Visual analytics tools can interpret drone images. This information can help reveal what areas climate change affects. It can also identify how changes are affecting different regions. Computer vision video annotation can analyze the annual changes in the color of vegetation. It gives insights into plant growth and drought risks.
Machine learning algorithms analyze environmental data to determine impacts on plant life. They also predict future changes based on past data sets. As a result, machines can better predict when events will occur and their effect on ecosystems.
Predicting Natural Disasters
Computer vision can analyze aerial images to identify potential natural disasters. Machine learning then uses this data to determine which patterns are significant. It predicts when and where disasters will occur more accurately than ever before.
Natural disasters like earthquakes and tsunamis have many telltale signs. For example, ground movement and water level changes are two signs. Computers can detect these events before they happen. Then, people have more time to prepare and evacuate safely.
How to Train Deep Learning Systems with Data Annotation
Labeling real-world objects and their values are essential for training deep learning models. Without labels, a machine learning system can't learn how to identify features. There's no way to tell what something is.
Annotating data involves creating a label for each piece of information. For instance, you might create one label for each area on the image where it's clear there is snow; this would be "snow." Suppose there are many types of snow present. (e.g., light vs. dark) You create separate labels for different types using labels like "light snow" and "dark snow."
Finally, you can train your model once you have all your labels. Training models involves feeding them all your annotated data. This way, they can learn what each label means and how it looks when paired with different images.
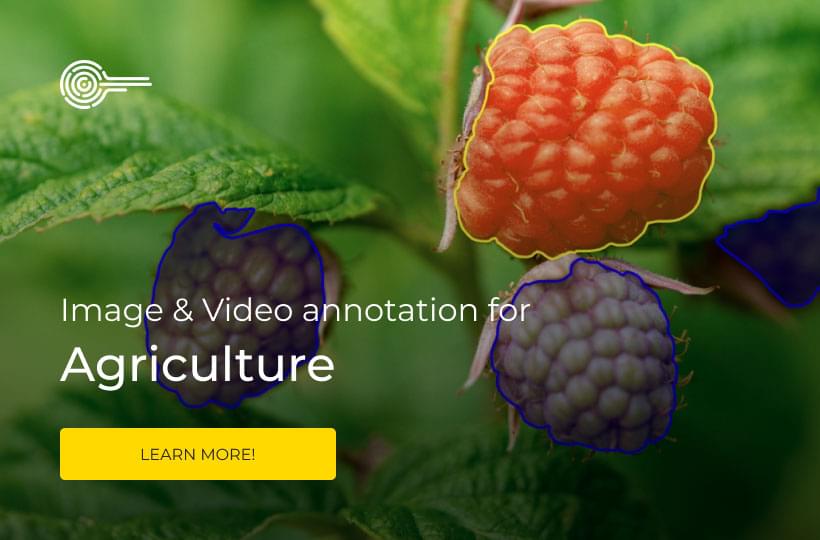
Conclusion
Many of the world's most pressing problems are complex and involve data. But the tools we have to analyze that data often aren't up to the task. Machine learning is one of the best ways we can use computers to understand climate change. It doesn't help the computer understand what's going on now.
Machine learning will also help predict future climate conditions. It can help find ways to adapt human behavior to mitigate or avoid adverse effects.Improve the quality of your model to make it more accurate. Keymakr provides all the tools you need to create high-quality computer vision video annotations. Using our annotation tools, you can annotate images and videos.