Choosing the Right Data Annotation Outsourcing Partner
Machine Learning (ML) projects often need from hundreds of thousands to millions of tagged data points to succeed. This is especially true in today's digital world, where AI and ML shape the future of companies. Outsourcing data annotation is helpful. Not only does it save time, but it can also be the difference between finishing a project in weeks instead of months.
Working with an annotation outsourcing partner doesn't just speed things up. It also ensures top-notch accuracy, security, and the needed flexibility. Because in-house teams might not always handle the big load of AI data labeling. So, outsourcing becomes more and more appealing.
When companies look into data annotation outsourcing, they seek more than just help. They want partners who speak the language of data. They look for experts who can guarantee superior accuracy and quality in the data tagged. This allows them to focus on what they do best and grow, while leaving the heavy lifting of data labeling to the pros.
Key Takeaways
- Understanding the expansive scale of data needed for successful AI models highlights the value of professional data annotation.
- Outsourcing to seasoned data annotators can drastically cut down on project time, enhancing market competitiveness.
- Data security protocols and specialized expertise are fundamental considerations when selecting an outsourcing partner.
- Efficient, flexible scaling options with outsourced data labeling can address fluctuating project demands.
- Accurate and quality-driven data annotation is synonymous with the assurance offered by expert external teams.
- Comparative analysis of cost structures and services is critical in choosing a data annotation partner aligned with your budget and project goals.

The Strategic Role of Data Annotation in AI Success
In today's fast-changing tech world, getting AI data labeling right is key to AI/ML projects doing well. With models and algorithms becoming advanced, the need for high-quality data labeling is growing. This shows why creating detailed and accurate training datasets is important.
Why Quality Data Annotation Is Essential for AI Models
Any AI/ML's success depends on the data it's built on – how good it is, how much there is, and if it's labeled correctly. Mistakes in labeling can throw off the entire model, making it give wrong results even with smart learning algorithms. So, quality data labeling is critical. It's what ensures AI does its job well, from customer service tech to complex healthcare diagnosis systems.
The Direct Link Between Data Annotation and AI Innovations
Great AI innovators like Amazon and Google put a lot into making sure their data is labeled accurately. Their focus on data labeling accuracy means they can provide top-notch, efficient solutions. This accuracy doesn't just help current systems; it sparks the creation of new tech that adapts to users and their needs, boosting efficiency in AI projects.
This careful approach to labeling data helps advance AI, enhancing everything from how cars drive themselves to improving how we get medical help.
Industry | Impact of Quality Data Annotation |
---|---|
Healthcare | Enables precise disease diagnosis and tailored treatment plans. |
Autonomous Vehicles | Improves navigation systems and object recognition for safer transportation. |
Retail | Enhances user experience through personalized product recommendations. |
Manufacturing | Aids in defect detection during quality control checks. |
Essentially, using high-quality data annotation strategically is crucial for making AI systems work well. It ensures these advanced tools meet their objectives, making data annotation a central success factor in AI projects.
Outsourcing Data Annotation: Boosting Efficiency and Quality
In AI development's intense world, using data labeling services boosts how projects work. It makes outcomes better. By outsourcing data annotation, working with large datasets for complex AI models becomes easier.
Enhanced quality data annotation becomes available when you outsource. This is key for big AI projects. Service providers like Keymakr use top-notch tools to annotate data accurately. This is critical for making machine learning models that work well. Moreover, they can quickly adjust their work to fit an AI project's changing needs, showing flexibility and speed.
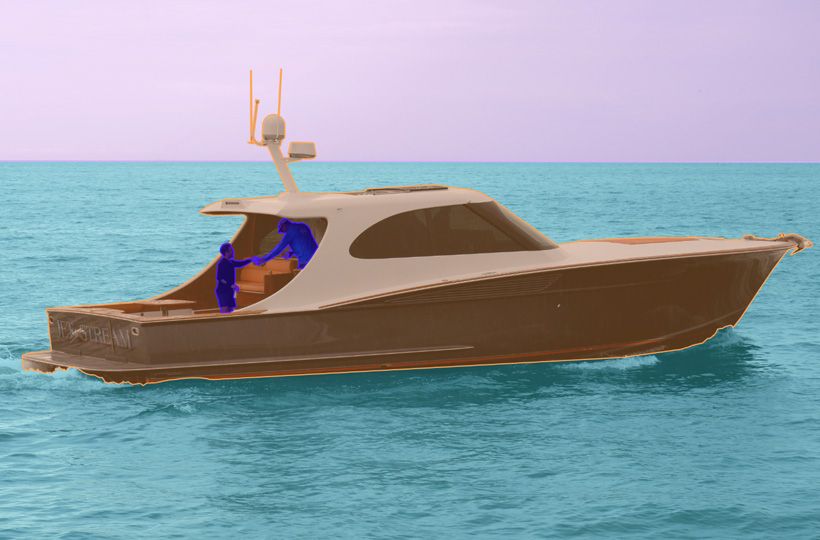
Here's how professional annotation services make a big difference:
- They often finish annotated data 50% quicker than teams inside can.
- Errors in data reduce by up to 40%, making machine learning models more reliable.
- Data labeling from outside can cut costs by 60% in AI projects.
By outsourcing, you let your team focus on making AI better, not handling data. This way, the project stays strong and flexible. External experts ensure data security and compliance, letting your team do what it does best.
Aspect | Internal Annotation | Outsourced Annotation |
---|---|---|
Time Efficiency | Limited by team size | Enhanced throughput with scalable teams |
Data Security | Dependent on internal protocols | Advanced security measures in place |
Cost Efficiency | High due to fixed overheads | Reduced costs due to optimized operations |
Quality of Data | Varies with team expertise | Consistently high with expert annotators |
So, outsourcing data annotation boosts both efficiency in AI projects and model quality. Choosing the right partner can lead your project to be more productive and innovative. Your AI solutions will stand out not just for being advanced but also for being ethical and cost-efficient.
Key Advantages of Outsourcing Data Annotation Services
Outsourcing data annotation helps create better AI models and offers big operational benefits. Working with expert data annotation services improves project results. This lets companies focus more on their main business goals. Exploring the benefits of a data annotation company shows how it can make projects better and more cost-effective.
Access to Specialized Expertise
Choosing a good data annotation company means you get experts in areas like medical imaging or understanding language. These experts know different data types and what they need for annotation. This is often too complex for teams within a company. By outsourcing, businesses can use this specialized knowledge. It leads to more accurate results and better AI performance.
Reducing Costs and Overheads
Outsourcing data annotation can cut costs and lower overheads significantly. Setting up an internal team for annotation is expensive. It includes hiring, training, and buying tech. Instead, outsourcing lets you use the tools and expertise of a data annotation company at a lesser cost. This can save you up to 40%.
Scaling Data Annotation Operations with Ease
Another big plus of outsourcing is how easily you can scale up. A good provider can add more resources when your project grows. This means you can handle big projects without hiring more people. It also avoids times when staff don’t have enough work.
Metric | In-House Annotation | Outsourced Annotation |
---|---|---|
Cost Efficiency | Higher initial & maintenance costs | Reduces costs by up to 40% |
Expertise Level | Limited to in-house skills | Access to specialized domain-expertise |
Scalability | Limited | Highly adaptable to demand |
Quality Assurance | Variable | Multi-tier quality checks ensuring >95% accuracy |
Turnaround Time | Dependent on in-house capabilities | Up to 50% faster completion rate |
Outsourcing gives you access to better tools and experts from around the world. It means faster, better projects. Working with an advanced data annotation company is more than just passing off tasks. It’s about boosting your AI efforts.
Ensuring Data Security and Privacy with External Partners
As more companies use AI and machine learning, keeping data privacy becomes key. This is especially true when companies outsource data labeling services. It's crucial to know how to keep data safe through the right steps.
When data is breached, it's not just about the money lost right away. It also hurts the company's reputation for a long time. On average, it takes 50 days to even find out about a breach. During this time, a lot of damage can be done. Outsourcing partners work hard to stop these issues by making sure data is as secure as possible.
Companies that outsource must make sure their partners follow global data laws. One major law is GDPR, which makes companies in Europe and beyond care for people's data very closely. Together, companies and their partners use special ways to guard data. This includes things like:
- Secure VPNs and encrypted data transfer,
- Advanced access controls and multi-factor authentication,
- Regular security audits and compliance checks.
Overall, outsourcing can make AI projects better but keeping data safe is crucial. Picking the right partner means choosing someone who is serious about following strict security rules. This helps businesses protect their data well, no matter how tech changes.
How to Evaluate a Data Annotation Company's Track Record
When thinking about outsourcing data annotation for AI and machine learning projects, it's crucial to look into the company's background. You need to check their expertise and how well they've performed in the past. This helps you pick a company that can meet your needs and standards.
Assessing Past Projects and Client Testimonials
Reviewing a company's previous projects on places like G2 shows their capabilities and the tasks they can manage. Find client testimonials to learn about their communication and ability to keep promises. Also, check if they have experience in handling big projects in data-heavy areas.
Industry-Specific Data Annotation Experience
Each industry has its unique data challenges and privacy concerns. It's important to choose a partner with knowledge of your field, be it healthcare, automotive, or finance. A good firm must prove they can handle your kind of projects with care and precision.
A company's credibility is also strengthened by certifications showing their commitment to quality and data security. These measures are essential in today's data-focused world. They help your operations run smoother and make your AI projects more effective when outsourcing data annotation.
Understanding the Cost Structures of Data Labeling Solutions
First off, where the service is located matters a lot. Companies in areas like Eastern Europe usually offer lower prices. This is because their costs to run the business are lower while they can still maintain a high level of professional expertise.
Knowing all the costs helps you budget well. It also avoids surprise expenses later on.
The way companies charge for data annotation affects how clear and valuable the service seems. They may charge by the hour, by dataset, or by the type of data. The complexity of the work also plays a role.
- On-Demand Flexibility: Hiring help only when you need it can save a lot of money. Instead of having a full-time team, you spend only when work is there, saving during slow times.
- Scalability: Outsourcing lets you easily add more resources when your projects get bigger. This is key for handling sudden increases in work.
- Quality Assurance: Good quality checks are essential for service providers. This can lower the costs linked to bad work, as it builds trust and avoids mistakes.
Technological Innovation in Outsourcing Data Annotation
In the field of artificial intelligence, the race for better data annotation boosts technology. Advanced data labeling tools such as our own proprietary Keylabs platform and innovative data annotation solutions have become key investments for many companies. Knowing how these technologies affect your AI work is very important.
The Importance of Scalability in AI Data Labeling
AI and ML technologies are always growing. The key to successful projects is the ability to handle different amounts of data. When you use scalability in outsourcing data annotation, you can cope with changing data needs. This is crucial for today's AI projects.
It's important to keep AI models effective by meeting their changing data needs. Being able to work with big data sets and smaller ones quickly is vital. Look at the facts: Outsourcing data annotation boosts AI's accuracy and speed. This keeps your project ahead and useful.
Outsourcing is a great option, especially when you can't do everything in-house. Beyond just the volume of data, the quality of data annotation is key for model performance. This is why scalability in outsourcing data annotation is so important.
- Flexible scaling avoids common hurdles for in-house teams with limited resources.
- It makes your budget use smarter, adapting to cost changes while managing data well.
- High data quality and security are maintained, even as data amounts increase.
When choosing a data labeling partner, think about future needs, too. It should help your project adapt over time. Knowing how to scale outsourcing lets your AI work stay nimble and accurate. It can adjust quickly as the data market changes.
Evaluating the Turnaround Time for Annotated Data Delivery
Improving AI/ML projects relies on getting annotated data quickly and accurately. Fast, precise data annotation does more than just get the project done. It also helps your product hit the market sooner with proper data labeling.
Setting Realistic Expectations for Project Timelines
It's key to agree on deadlines early when you outsource annotation. This keeps the project moving smoothly. A good annotation service will not only promise realistic deadlines but will also meet them without fail. This keeps your AI projects running smoothly, avoiding delays and extra costs.
In short, when selecting efficient data annotation services, look closely at their timeliness and security promises. This check will ensure they can handle your project efficiently. It will also improve your product’s launch readiness with great data labeling.
Summary
Today, the success of AI/ML projects depends on accurate data annotation. Many companies are choosing to outsource this task. They see it as a way to gain expertise and save money while keeping flexible in a competitive market.
Vendors like Keymakr offer specialized knowledge for better services. For example, they can make e-commerce recommendations or healthcare diagnosis more efficient and effective. By partnering with these experts, businesses can focus on growth and development.
FAQ
Why is choosing the right outsourcing data annotation partner crucial for AI success?
The right partner is key for quality AI data labeling. They have the skills and experience to boost AI/ML model performance and innovation. This gives your business a competitive edge in the market.
How does the quality of data annotation directly affect AI innovation?
Good data labeling ensures AI models understand data accurately. This leads to more trustworthy predictions or actions. It also speeds up AI innovation, adding more value to your business.
What are the benefits of outsourcing data annotation in terms of efficiency and quality?
Outsourcing can improve the quality of data labeling. Partners bring expertise and use the latest tools for better accuracy. They also make AI projects more efficient by quickly adjusting to project needs.
What specific expertise do outsourcing data annotation companies offer?
They are experts in labeling image, video, and text data. They use advanced tools to meet your industry's unique needs. This results in accurate and high-quality labeling.
How can outsourcing data annotation services reduce costs and overheads?
Outsourcing saves you from the costs of an in-house team and tools. This means you spend less while benefiting from expert services. It's a smart move for many businesses.
How does an external data annotation partner help scale operations?
A good outsourcing partner can quickly adjust to your data labeling needs. They maintain quality even when handling more or less data. This flexibility is vital for project success.
What measures do data annotation outsourcing services take to ensure data security and privacy?
They use strong security measures like data encryption and GDPR compliance. Some also offer on-premises options. These steps ensure your data is safe and handled with care.
What criteria should be used to evaluate a data annotation company's track record?
Look at their past work, what clients say, and how they meet project deadlines. Their experience in your field and the tools they use are also important. This shows their credibility.
Why is it important to understand an annotation service provider's cost structure?
Understanding the costs helps your budget and prevents surprise expenses. It ensures transparency in the partnership. This knowledge makes your collaboration more effective and worry-free.
How do advanced tools and technologies influence the quality of data annotation?
Better tools and methods make data labeling more accurate and faster. They can also use AI to assist, ensuring the data is of top quality. This all helps train AI models better.
Can outsourcing data annotation adapt to the changing requirements of AI projects?
Yes, outsourcing partners can quickly adjust to any data labeling needs. They can grow or shrink their work based on your project's stage and goals. This flexibility is crucial for project success.
How does the speed of data annotation affect a project's market readiness?
Quick data annotation means AI models are ready for the market faster. This lets your business seize market chances quickly. It also helps you plan your project timeline better and launch sooner.
Are there outsourcing options for both image annotation and text annotation services?
Yes, companies offer both image and text annotation services. They create custom labeling solutions for different data types. This flexibility meets the various needs of AI/ML projects.
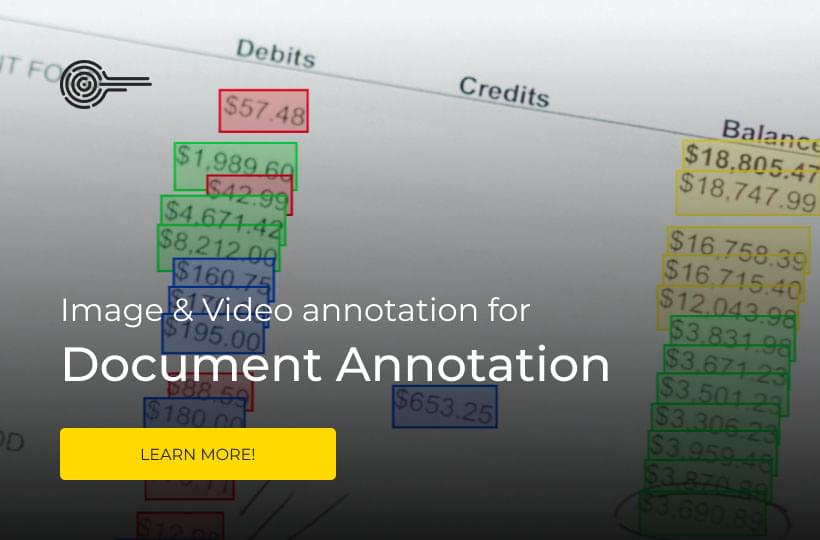