Boosting Manufacturing Efficiency with Image and Video Annotation

Manufacturing data annotation services play a critical role in machine learning and AI. They are essential for improving various quality control processes. By using precise image annotation and video annotation, companies can train AI systems to recognize objects and automate tasks. This leads to faster, more accurate, and scalable production lines.
AI in manufacturing and industrial automation can cut costs and boost efficiency. It does this through data analysis, increasing productivity, reducing waste, and improving operational speed and reliability. Keymakr, with over 600 trained experts, offers high-quality annotation services. These services are vital for manufacturers looking to enhance their AI solutions. Sophisticated computer vision algorithms help streamline operations, improve quality control, and achieve superior manufacturing efficiency.
Key Takeaways
- Accurate image annotation and video annotation are essential for machine learning and AI solutions in manufacturing.
- Advanced annotation services significantly enhance operational speed, quality control, and overall productivity.
- Computer vision algorithms optimize inventory management and reduce waste.
- AI-powered defect detection and quality control ensure high product standards.

Understanding Manufacturing Data Annotation Services
Data annotation services are key to AI's success in manufacturing. Experts recognize the importance of annotating data, whether manually or through automated means. This process is vital for training AI models to understand and interpret data. It enables AI to operate more efficiently and intelligently in manufacturing settings.
What is Data Annotation?
Data annotation labels data like images, videos, text, or audio for AI models. This labeled data is the basis for training machine learning algorithms. It allows them to make decisions, such as spotting defects or predicting maintenance needs. Both manual and automated annotation methods are used to enhance AI training.
Importance in Manufacturing
Robust data annotation services are essential for AI in manufacturing. Precise data labeling enables AI models to perform tasks more accurately and efficiently. This leads to several benefits:
- Production Speed: Robotics data annotation has led to a 20% increase in production speed.
- Error Reduction: A 30% decrease in manufacturing errors was observed.
- Operational Efficiency: Advanced annotation in e-commerce improved packing speed by 40% and reduced product damage by 50%.
Types of Data Annotations
Knowing the different types of annotations is vital for successful AI in manufacturing:
- Image Annotations: Essential for image recognition in self-driving cars and medical diagnostics.
- Text Annotations: Crucial for natural language processing, sentiment analysis, and language translation tasks.
- Audio Annotations: Necessary for training speech recognition models in virtual assistants.
- Video Annotations: Used extensively in security systems and autonomous vehicles for object tracking and action labeling.
Each annotation type is critical for improving manufacturing precision, efficiency, and productivity. Well-annotated data significantly impacts AI performance. The right combination of manual and automated annotations ensures thorough training and ongoing AI improvement.
Benefits of Image and Video Annotation
In recent years, the advantages of video annotation have become clear, mainly in manufacturing. Advanced data annotation boosts precision, automates processes, and streamlines quality control. This is vital for businesses aiming to stay ahead in a fast-changing market.
Enhanced Precision in Operations
Image and video annotation greatly enhance manufacturing precision. They provide detailed, accurate data for machine training, cutting down on errors and downtime. Statistics show a 51% increase in Asia-Pacific organizations using AI-/ML models, from 37% in 2020. This highlights data annotation's key role across industries.
For instance, car makers use image annotation for autonomous driving. They segment road objects for efficient navigation. This precision is not exclusive to automotive. In healthcare, polygon techniques annotate human organs for early disease detection. This leads to more accurate diagnoses and cost savings on expensive scanners.
- Reducing operational errors
- Minimizing downtime
- Optimizing machinery performance
Video annotation's advantages extend beyond automotive and healthcare. In retail, bounding box labeling tracks products. In security and surveillance, it prevents serious crimes by analyzing behaviors and identifying faces in crowds.
Streamlined Quality Control Processes
Video annotation also automates quality control, ensuring consistent product quality and adherence to standards. Keymakr, for example, boasts a 98% accuracy rate in its annotation services. It offers ready-to-deploy experts and AI tools for faster, more efficient work. Such automation is critical for industries like retail and security, where it prevents crimes by analyzing behaviors and identifying faces.
- Consistent product quality
- Compliance with industry standards
- Enhanced efficiency in inspection processes
Key Applications in Manufacturing
In manufacturing, image and video annotation services are transforming efficiency. They are key in object detection for quality checks and automating assembly lines. These advancements are driven by AI applications in manufacturing.
Object Detection for Quality Assurance
Object detection is essential for quality assurance in manufacturing. Advanced AI models quickly spot defects or issues in products. This ensures only high-quality items move through the supply chain.
AI applications, like bounding boxes, accurately identify defects. This rigorous quality control is vital for maintaining brand reputation and reducing waste.
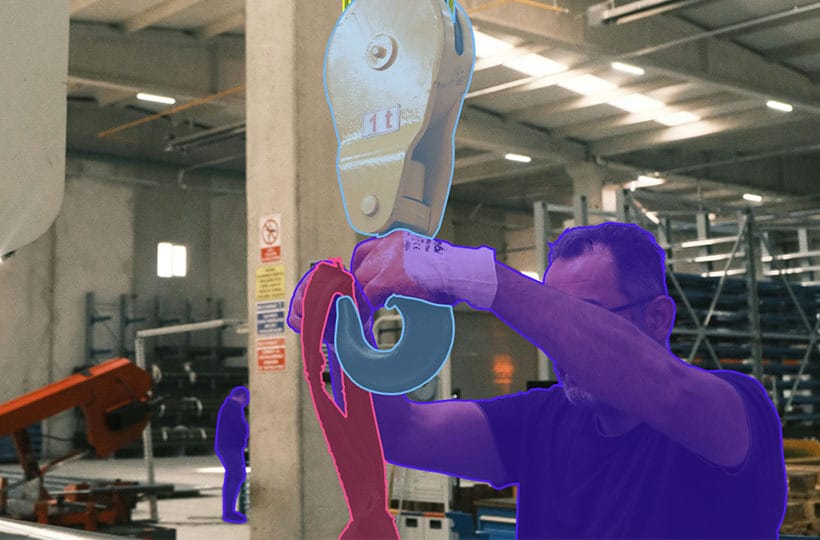
Automation in Assembly Lines
Automated assembly lines, powered by video annotation tools, have revolutionized manufacturing. These systems reduce manual intervention, boosting efficiency and throughput. AI applications in manufacturing support these lines, ensuring smooth operation.
Video annotation trains AI models for complex tasks. This enhances robotic system performance. 3D cuboids enable machines to handle objects in three-dimensional space, improving precision and reliability.
“High-quality, precisely annotated datasets are critical for enabling various AI applications in manufacturing, leading to unprecedented accuracy and efficiency in production workflows.”
Choosing the Right Annotation Service Provider
When picking the right annotation service provider, several factors must be considered. A thorough evaluation ensures the chosen partner meets specific needs, boosting AI success. This part explores key considerations and the role of expertise and experience in making a wise choice.
Factors to Consider
Assessing key factors is vital for project success when selecting annotation services:
- Accuracy and Speed: Ensure the provider maintains high precision and annotates quickly.
- Scalability: Check if they can handle large datasets without quality drops.
- Cost-Effectiveness: Match the pricing with your project goals for the best ROI.
- Security Measures: Confirm strong data protection systems are in place.
Working with providers like Keymakr ensures adherence to data privacy and cybersecurity standards. This is essential for client trust and data integrity.
Integration of AI in Manufacturing
The AI integration in manufacturing involves embedding intelligent systems that can learn and adapt. Companies like Siemens and General Electric are at the forefront, using AI to improve product quality and enable real-time monitoring. Their goal is to boost operational efficiency and enhance product design.
AI-driven predictive analytics, manufacturers can predict equipment failure and manage inventory and demand better.
Impact on Data Processing Efficiency
The AI impact on data efficiency is significant, given the vast data generated in manufacturing. AI technologies like computer vision and context-aware computing are key in quality control, allowing for precise identification of defective products. AI also improves energy management by reducing wastage and cutting costs.
Reports show that companies using machine learning in manufacturing see a significant increase in productivity and a decrease in downtime. This is due to data-driven insights.
Real-time data aggregation and analysis give manufacturers a competitive edge, enabling them to quickly respond to market changes. Yet, achieving this efficiency requires a highly skilled workforce and expert annotation services.
Common Challenges in Data Annotation
In the manufacturing sector, tackling data annotation hurdles is key to successful AI adoption. These obstacles include ensuring data precision and managing volume, both critical for AI model training and deployment.
Ensuring Consistency and Accuracy
High-quality datasets rely on strict ensuring data accuracy and consistency. The subjective nature of annotation can introduce labeling inconsistencies. In MilTech, where data security is a top priority, accuracy is even more essential. To overcome these issues, quality assurance (QA) processes are vital. These include continuous collaboration and manual checks.
Research shows that overreliance on automation can exacerbate problems. A blend of human and automated methods is recommended for precise labeling.
Managing Large Volumes of Data
Managing vast datasets poses significant challenges for manufacturing firms. Robust infrastructure and advanced data processing tools are needed for timely AI implementation. Manual annotation, though ensuring quality, is slow and resource-heavy.
Semi-automatic methods, combining human input with Large Language Models (LLMs), offer a solution. They balance accuracy with scalability. For efficient AI/ML projects, large annotated datasets are essential. Companies must strategize to handle these tasks efficiently.
Best Practices for Data Annotation
In the world of data annotation, it's vital to have clear guidelines and to check quality regularly. Following data annotation best practices ensures annotations are consistent and reliable. This boosts the performance of machine learning models in manufacturing.
Setting Clear Guidelines
Creating detailed annotation guidelines is key for consistency in datasets. These guidelines outline the scope, rules, and standards for annotation tasks. They ensure all annotators understand the project's requirements.
- Define Specific Criteria: Make sure annotators know what to tag and how to do it.
- Standardize Annotations: Use the same labels and bounding boxes for consistency.
- Include Examples: Show visual examples to demonstrate proper annotation techniques.
Regular Quality Checks
Regular quality checks in annotation are essential to ensure data accuracy. This involves several verification steps to catch and fix errors quickly.
- Peer Review: Regularly check each annotator's work against guidelines.
- Automated Testing: Use tools to spot and flag any discrepancies.
- Continuous Feedback: Create a feedback loop for annotators to learn from mistakes and get better.
By following these data annotation best practices, including clear annotation guidelines and regular quality checks in annotation, you can significantly lower errors. This will also enhance the effectiveness of AI models.
Future Trends in Manufacturing Data Annotation
As we explore the future of manufacturing data annotation, several trends are shaping the industry. The integration of IoT and predictive analytics is set to revolutionize how we process and use manufacturing data.
The Impact of IoT on Data Needs
The Internet of Things (IoT) has significantly increased data needs in manufacturing. IoT devices produce vast amounts of data every second. Advanced annotation techniques are needed to make this data useful. Multi-modal annotation is becoming key to handle data from images, text, audio, and video.
IoT integration with annotation processes ensures real-time data use. This leads to better responsiveness and efficiency in manufacturing operations. Privacy-preserving annotation techniques like differential privacy and federated learning are also being adopted. This is essential as data volume grows, projected to reach 175ZB by 2025, with most being unstructured.
Predictive Analytics and Maintenance
Predictive analytics in manufacturing is gaining traction. It aims to reduce downtime and improve operational agility. Advanced data annotation methods enable predictive maintenance to foresee equipment failures. This reduces unexpected downtime and maintenance costs, giving a competitive edge.
Automated annotation tools are expected to boost efficiency by cutting manual labor costs. Ensuring quality and mitigating bias in annotated datasets is critical for predictive model effectiveness. Collaborative annotation platforms enhance real-time collaboration and quality assurance, improving predictive analytics reliability.
Trend | Impact |
---|---|
IoT Integration | Enhanced real-time data processing and operational efficiency |
Predictive Analytics | Reduced downtime, improved maintenance, and cost savings |
Automated Annotation Tools | Increased efficiency, reduced manual efforts |
Privacy-preserving Annotation | Addressed data privacy concerns |
Collaborative Annotation Platforms | Improved quality assurance and real-time collaboration |
Getting Started with Manufacturing Data Annotation
Starting a manufacturing data annotation project requires several key steps for success. First, we must clearly define the project's scope. This means deciding on the type of data, whether it's images, videos, or text, that needs to be annotated. It's also important to understand the format and detail level required for setting project parameters accurately. High-quality data collection from the start is essential for reliable results.
Steps to Initiate a Project
Initiating a data annotation project demands a structured approach. Essential steps include:
- Defining the project scope and objectives.
- Selecting the right tools and technologies, considering factors such as user interface, annotation capabilities, and collaboration features.
- Training annotators to ensure they are skilled and proficient, setting quality control processes to maintain accuracy.
Implementing regular quality checks using both manual and automated methods is also vital. This helps maintain the precision and reliability of annotations. These steps are critical, given the need for efficiency and scalability when handling large datasets.
Collaborating with an Annotation Partner
Collaborating with an experienced annotation partner is essential for adapting the process to specific manufacturing needs. They bring specialized expertise in annotations tailored for manufacturing applications like quality control and automation. They also offer advanced AI services to boost operational efficiency. Working together ensures ethical practices, transparency, and compliance, meeting the manufacturing industry's standards.
By partnering with experts and following these structured steps, we can overcome the challenges of starting with data annotation. This ensures high accuracy and consistency, which are critical for deploying machine learning and AI in manufacturing.
FAQ
What is Data Annotation?
Data annotation is the process of labeling data, like images or videos, to help AI models understand it. This step is vital for training AI to handle various manufacturing tasks effectively.
Why is data annotation important in manufacturing?
In manufacturing, data annotation boosts efficiency by allowing AI to recognize objects and automate tasks. This leads to faster, more accurate, and scalable production lines.
What are the types of data annotations used in manufacturing?
Manufacturing uses several data annotation types, including image, text, audio, and video annotations. Each type enhances machine learning models, improving efficiency and productivity.
How does image and video annotation enhance manufacturing precision?
Image and video annotation improve precision by creating detailed training datasets for machines. This results in fewer errors and less downtime.
How do automated quality control processes benefit from video annotation?
Automated video analysis streamlines quality control, ensuring consistent product quality and adherence to standards. This optimizes inspection and monitoring processes significantly.
What are the key applications of image and video annotation in manufacturing?
Key applications include object detection for quality assurance and automating assembly lines. These reduce manual intervention and increase production speed.
What factors should be considered when choosing a data annotation service provider?
Important factors include accuracy, speed, scalability, cost-effectiveness, and the provider's manufacturing expertise. These ensure the best fit for specific needs.
How do AI and machine learning integrate into manufacturing processes?
AI and machine learning transform manufacturing through automation and predictive analytics. They enhance data processing, enable real-time decisions, and promote proactive maintenance.
What are common challenges in manufacturing data annotation?
Challenges include maintaining data consistency and accuracy, and efficiently managing large datasets. Robust infrastructure and advanced tools are necessary to overcome these hurdles.
How are pricing models for annotation services structured?
Pricing models vary, with options like fixed rates and flexible pricing based on project complexity. Understanding these models helps manufacturers budget and choose the most cost-effective services.
What are the best practices for data annotation in manufacturing?
Best practices include setting clear guidelines for data consistency and conducting regular quality checks. These practices reduce errors and enhance machine learning model reliability.
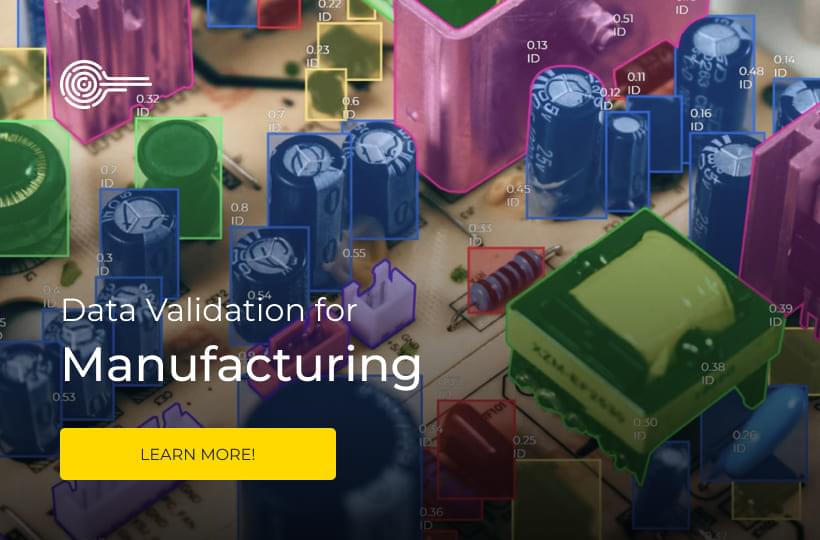