Boost Your Annotation Accuracy with These Pro Tips
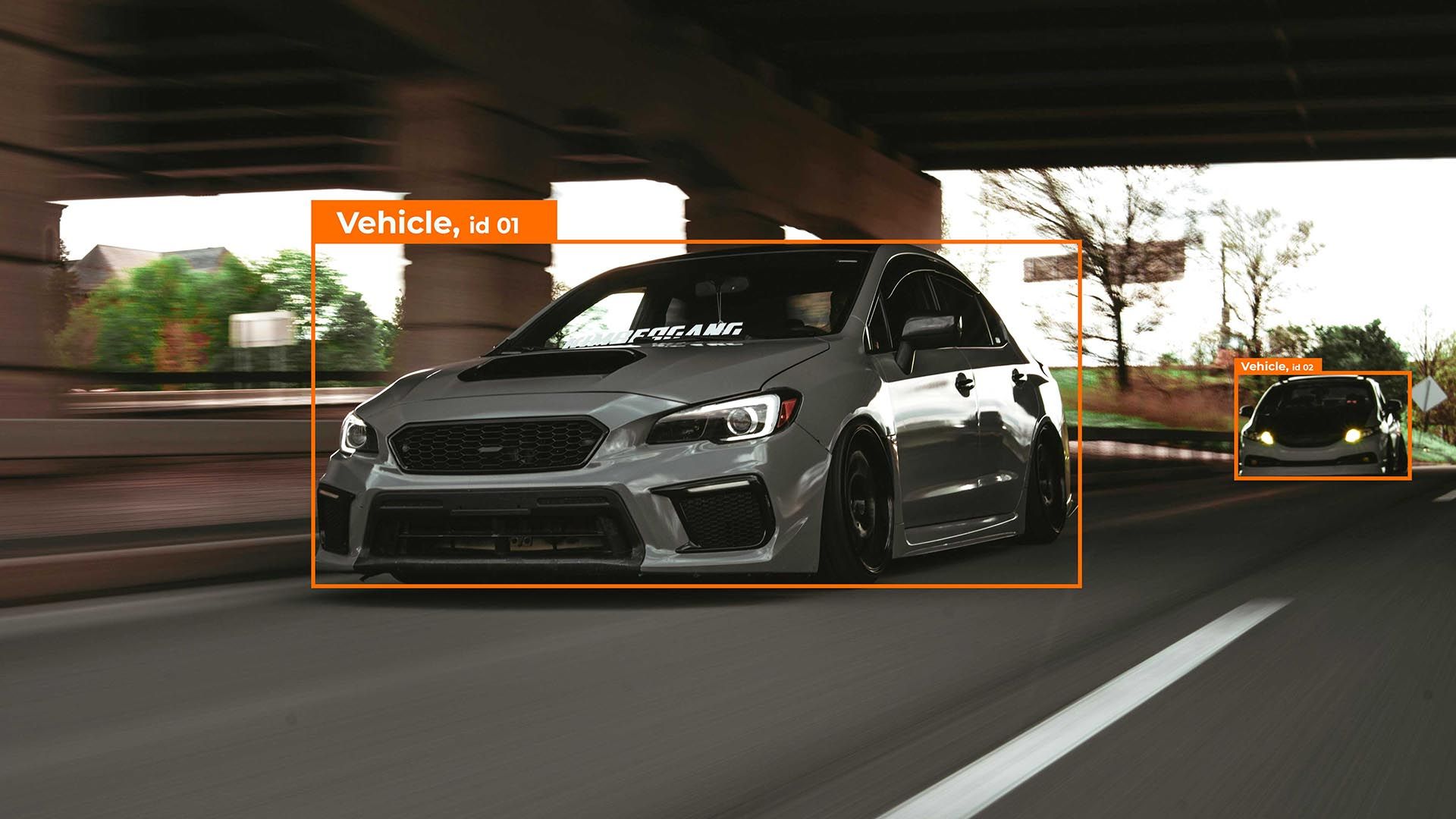
Annotation is essential for AI and ML, turning data into something machines can learn from. The key is not how much you annotate, but how carefully. Good tagging leads to accurate predictions by machine learning models. To improve your tagging's accuracy, set clear goals and guidelines. This makes everything more consistent and helps users and decision-makers.
AI tools, like those from Keylabs, are changing the game, making tagging data both quicker and more cost-effective. They use a mix of AI and human skill to do this. By focusing on tagging precision, you're not just improving algorithms. You're also helping with personalized health care, safer self-driving cars, and instant translations.
Key Takeaways
- Understand the gravity of accurate annotations in data labeling to support the efficiency and integrity of AI projects.
- Implement clear annotation guidelines for consistent and precise data labeling.
- Utilize advanced annotation platforms and AI-enhanced tools for efficient and accurate data processing.
- Recognize the critical role of diversity in data sets to prevent bias and promote model resilience.
- Ensure ongoing communication and feedback to foster continuous improvement in data annotation projects.
- Place a premium on data privacy and quality control, particularly in sensitive industries where precision is non-negotiable.

The Pivotal Role of Data Annotation in AI and ML
In the fast-changing world of Artificial Intelligence (AI) and Machine Learning (ML), annotating data is crucial. Good data annotation helps train AI to be more accurate. It makes AI work better by understanding patterns and new information well.
Understanding the Basics of Data Labeling
Data labeling is adding labels to data so machines can understand it. This is vital in areas like self-driving cars and healthcare. It makes AI do its job more accurately. Plus, it improves how well AI works in different tasks.
Quality: The Cornerstone of Machine Learning Models
How good AI and ML models are depends on the data they learn from. Top-notch labeling leads to models we can trust, especially in important tasks. Using the best practices in annotations is key to making models work well.
The data annotation market is growing fast, set to hit $6.45 billion by 2027. This huge growth shows the need for carefully annotated data. It ensures AI keeps getting smarter and more useful.
Industry | Annotation Needs | Impact of Quality Annotation |
---|---|---|
Autonomous Vehicles | Daily dataset refresh | Enhanced navigation and safety protocols |
Healthcare | Monthly dataset updates | Improved diagnostic accuracy and patient outcomes |
Finance | High-frequency labeling accuracy | Better fraud detection and risk management |
Data annotation is key to making AI available to everyone. It improves how accurate and useful AI is across many fields. Making data labeling more accurate and of high quality is very important. It's a must-do step.
Unveiling the Impacts of Data Annotation Errors
In the world of artificial intelligence and machine learning, data annotation plays a critical role. Using efficient data annotation strategies is key for model accuracy. It helps improve automation and decision-making in our daily lives. But, if the data annotation is wrong, it causes big problems. This leads to errors in AI decisions, especially in fields like healthcare and self-driving cars.
Incorrect data annotation leads to mistakes in the machine learning process. This can result in wrong or biased predictions. Imagine a wrong label on a medical image, leading to a misdiagnosis. Or, flawed data on road conditions, making autonomous vehicles drive unsafely. These errors can risk people's lives.
To make machine learning safer and more accurate, we must focus on being precise. The trend is to use AI and automation to annotate data. This makes the process fast, precise, and cost-effective. Let's look closer at how these new methods help in data annotation:
Trend | Description | Impact on Annotation Quality |
---|---|---|
AI-driven Annotation | Utilization of AI to automate data labeling tasks, reducing human error. | Increases accuracy and speed, reducing costs associated with manual annotation. |
Multi-modal Annotation | Annotation of various data types like images, text, audio, and video within a single dataset. | Enhances model performance by providing comprehensive multi-contextual data insights. |
Collaborative Platforms | Technological platforms that allow distributed teams to label data collaboratively in real-time. | Improves consistency and allows for real-time problem-solving and error correction. |
Quality Control Measures | Implementing regular checks and audits of the annotated data. | Ensures ongoing accuracy, uniformity, and fairness in data labeling across projects. |
So, by using efficient data annotation strategies, we can avoid the consequences of inaccurate data annotation. These methods ensure AI operates well in many areas. This leads to innovative and trustworthy technology.
Annotation Accuracy Improvement Tips
Want to make your machine learning projects better by improving data annotation? By following certain steps, you can make your annotations more accurate. This is key for strong AI applications.
Streamlining the Labeling Process
To make your data annotation better, you must simplify the process. Start by setting clear guidelines for everyone to follow. This makes sure all annotators know what to do, cutting down on mistakes and making your annotations more precise.
Use tools like Keylabs. They are precise and help you make detailed annotations. Choose annotation tools with easy-to-use designs. This makes labeling and organization faster and more accurate. Simple tools cut down on confusion, making annotation quicker and more exact.
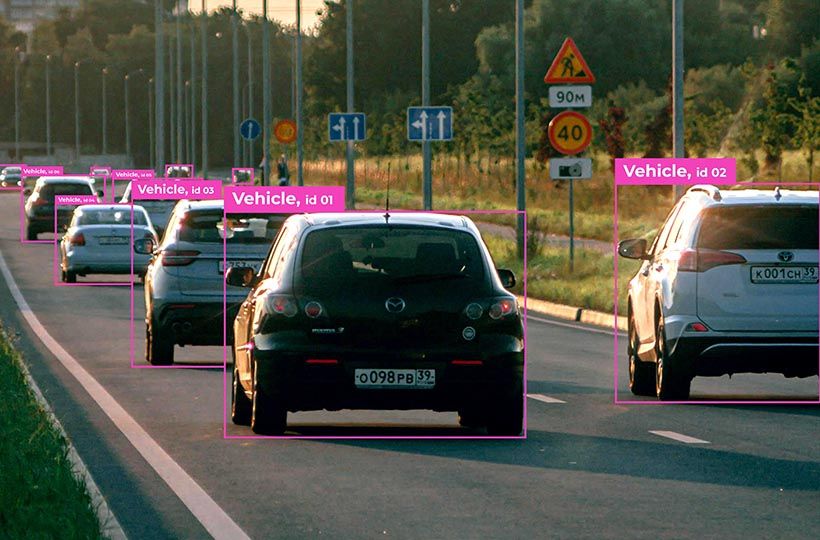
Setting Up Systematic Review Cycles
Checking annotations regularly is important for high quality. Have a system where different people review and compare annotations. This uncovers mistakes and ensures everyone is on the same page.
Make sure there’s feedback for the annotators. This helps them improve, making your process better over time.
By following these methods and promoting learning in your team, you’ll notice a big change in your data’s accuracy. This is crucial for your AI systems to do well in real-life scenarios.
Emphasizing Annotation Consistency Across Datasets
For AI to learn well, we must keep annotations the same across datasets. Using techniques for enhancing annotation consistency makes training better. It also boosts AI's effectiveness in real-life tasks.
Optimizing annotated data means picking annotators who are skilled. They make our data top-notch with their expertise. To keep things consistent and accurate, we must check the data carefully as it's annotated.
Advanced annotation tools help a lot in this area. They have features that guide annotators to keep things consistent. This makes data better for AI's complex jobs. Also, by continually updating the guidelines with the latest AI info, we make annotations even more accurate.
Keeping data quality high isn't a one-time job. It requires ongoing checks and improvements. This work is key in making AI models work better in the real world.
Tools like those from Encord can help. They offer micro-models and AI-powered labeling tools. These can make the annotation process more precise and consistent. This, in turn, boosts the value of the datasets.
To sum up, it's crucial to use strong techniques for enhancing annotation consistency. With solid, consistent data, AI systems can tackle real challenges better. This leads to insights that are more reliable and useful.
Implementing Quality Control in Annotation Workflows
Today, it's crucial to focus on enhancing annotation quality for better machine learning models. By improving annotation accuracy, we ensure our data is top-notch. This lets us use annotators' skills to make our models better.
Maintaining High Standards of Data Precision
Creating reliable annotated data starts with strict quality standards. We use rigorous stats to check annotations and spot errors early. Using tools like Keylabs once again helps a lot by making labeling more accurate, improving data quality.
The Role of Annotator Expertise and Feedback
Skilled annotators improve the process a lot. They make sure the data is interpreted correctly, helping machines learn better. Structured feedback is also important. It helps keep annotations accurate. Human-in-the-loop labeling combines human judgment and automated efficiency. This reduces errors and boosts algorithm training.
Check out the table below for more on how to keep annotation accuracy high:
Quality Control Element | Description | Impact on Annotation Accuracy |
---|---|---|
Advanced Labeling Software | Uses tools like Keylabs tailored to diverse dataset types. | Enhances overall labeling accuracy. |
Iterative Training | Continuous training cycles that incorporate annotator feedback. | Leads to improved data labeling and reduced errors. |
Expert Annotators | Well-trained professionals experienced in data interpretation. | High data accuracy, leading to better machine learning models. |
Human-in-the-Loop | Combines automated tools with human verification. | Optimal balance between speed and precision. |
By always improving how we do things, we make our data more reliable. This helps build AI models that work great and provide helpful insights.
Strategies for Efficient Data Annotation
Creating efficient data annotation strategies is vital for businesses wanting to use machine learning (ML) and artificial intelligence (AI). Knowing how to make improving labeling accuracy a goal is key. You need to understand strategic steps and helpful tips to make your data tagging more precise.
Establishing a strategic annotation framework comes first. This plan should match your ML goals and the data you need. A clear strategy makes the tagging process smoother and improves the data's quality. It ensures your team knows exactly what to do, cutting down on errors.
Starting small is wise if you're new to these strategies. It lets you fix any problems without using too many resources or money. Also, consider your budget carefully. It should cover all costs so you can spend wisely on tools, technology, and annotators.
Component | Importance | Strategy |
---|---|---|
Human Expertise | Crucial for quality | Integrate skilled annotators with AI tools |
Technology | Enhances efficiency | Use advanced annotation tools |
Quality Assurance | Ensures data integrity | Regular reviews and audits |
Scalability | Supports growth | Design processes to expand seamlessly |
Also, using active learning techniques can speed up data tagging. This method, called human-in-the-loop, combines human judgment and ML accuracy. It ensures tagging is quicker and more precise, even with complex data.
Improving labeling accuracy is all about careful planning and using technology and human power wisely. By following these efficient data annotation strategies, companies can build datasets they can trust. This drives the success of their AI and ML projects.
Optimizing Annotated Data for Maximum Utility in AI
In the field of artificial intelligence, using annotated data well is key. It helps algorithms train and work better. To make data truly useful, we need accurate data labeling precision techniques. Also, we must keep data well-preserved for years to come.
Leveraging Annotated Data for Algorithm Training
Using annotated data to train AI models requires great care. For example, integrating tools like GPT-4 and Gemini into your workflows can help by making data labeling faster and more accurate. This cuts down on the time people spend doing it manually.
Techniques for Enhancing Annotation Consistency
Using techniques for enhancing annotation consistency makes sure data is correct and the same across many sets. It all starts by making clear rules for how to annotate. This makes a standard everyone can follow, which keeps things consistent.
Consistent data annotation boosts data quality. It also makes AI models better and more reliable.
To make annotations even better over time, teams should review their work often. Getting feedback helps fix any mistakes in annotations. It also makes sure everything is meeting the project's goals. These review sessions are key points for team learning and improvement.
- Define clear annotation guidelines
- Utilize consistent labeling formats
- Incorporate feedback during iterative processes
Establishing inter-annotator agreement is also important. It helps set a standard for all annotators to follow. This way, their results are similar. This reduces mistakes and gives more trustable data for AI goals.
In sum, using these techniques for enhancing annotation consistency greatly boosts the quality and speed of data annotation. This success leads to better AI model performance for organizations.
Adopting Advanced Tools for Improved Labeling Accuracy
In the world of Artificial Intelligence (AI) and Machine Learning (ML), getting data right is key. For the best model performance, you need big datasets with thousands of elements. According to MIT, even the best datasets can have a 3.4% error in labels. This shows how important it is to carefully annotate data for good AI performance.
Utilization of Image Annotation Software
In areas like healthcare and automotive, precision is essential and can save lives. Here, using advanced tools, such as image annotation software, is vital. These tools, together with skilled annotators and proper training, boost the accuracy of labeling. They can improve an algorithm's accuracy by more than 20%.
Exploring Open-Source Annotation Tools for Budget-Friendly Accuracy
If you're looking to save money without losing quality, open-source tools are a good choice. Keylabs also offers tools for auto-labeling with the help of AI. This approach combines innovation with cost-effectiveness. It's especially useful for detailed tasks like LiDAR and polygon annotation. These are crucial in tech areas from city planning to self-driving cars.
FAQ
How does data annotation impact AI and ML?
Data annotation is key for AI and ML. Accurate labeling improves algorithm learning. This leads to better predictions in varied fields.
Why is quality important in building machine learning models?
Quality data annotation is critical for ML models. Good annotations help models work well in real scenarios. This lowers error risks and boosts performance.
What are the consequences of inaccurate data annotation?
Bad annotations can cripple model performance and trust. It can cause wrong predictions and biased decisions, posing ethical issues.
How can streamlining the labeling process improve annotation accuracy?
Efficient workflows and effective tools lessen errors. They enhance the quality of annotations.
What is the importance of maintaining annotation consistency across datasets?
Consistent annotations are key for AI to learn correctly from varied data. They ensure smooth model training and prevent algorithm confusion.
What are the best practices for text annotation?
For text, strict guidelines and specific techniques are essential. It's important to update methods and criteria as needed.
What constitutes effective quality control in annotation workflows?
Good quality control means setting high standards and validating annotations statistically. It relies on annotator skills and feedback to weed out mistakes.
How can efficiency be achieved in data annotation?
Specialized services and tech tools can make annotation more efficient. A well-planned annotation method helps save time and resources.
What are some strategies to ensure data utility and reusability in AI?
Format data properly, document the annotation process, and ensure data preservation. This increases the data's life and usefulness for future AI work.
How can advanced tools improve labeling accuracy?
Advanced tools, like image annotation software, bolster labeling accuracy. They offer features that make visual data annotations for AI, like computer vision, more precise and consistent.
Are there budget-friendly options for accurate data annotation tools?
Yes, there are open-source tools that are budget-friendly. They come with strong features and a supportive community, ensuring quality annotations without high costs.
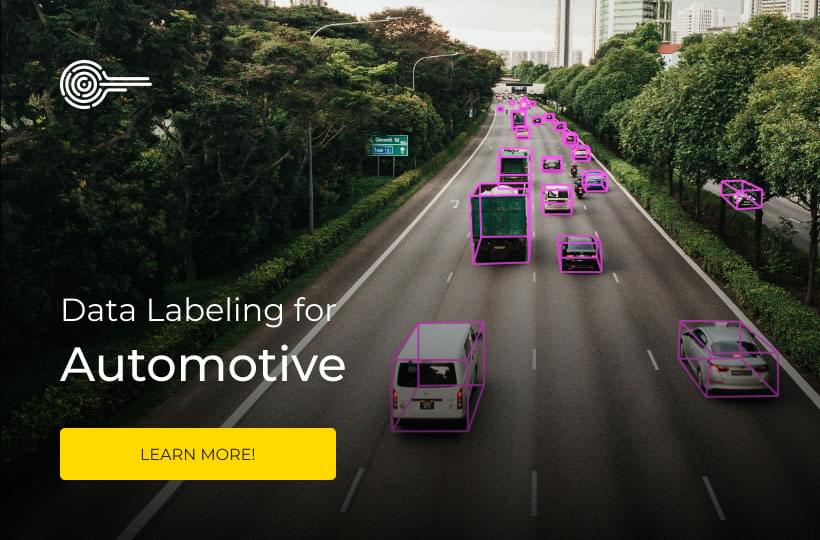