Beyond Clicks: Data Annotation for AI-powered Customer Segmentation
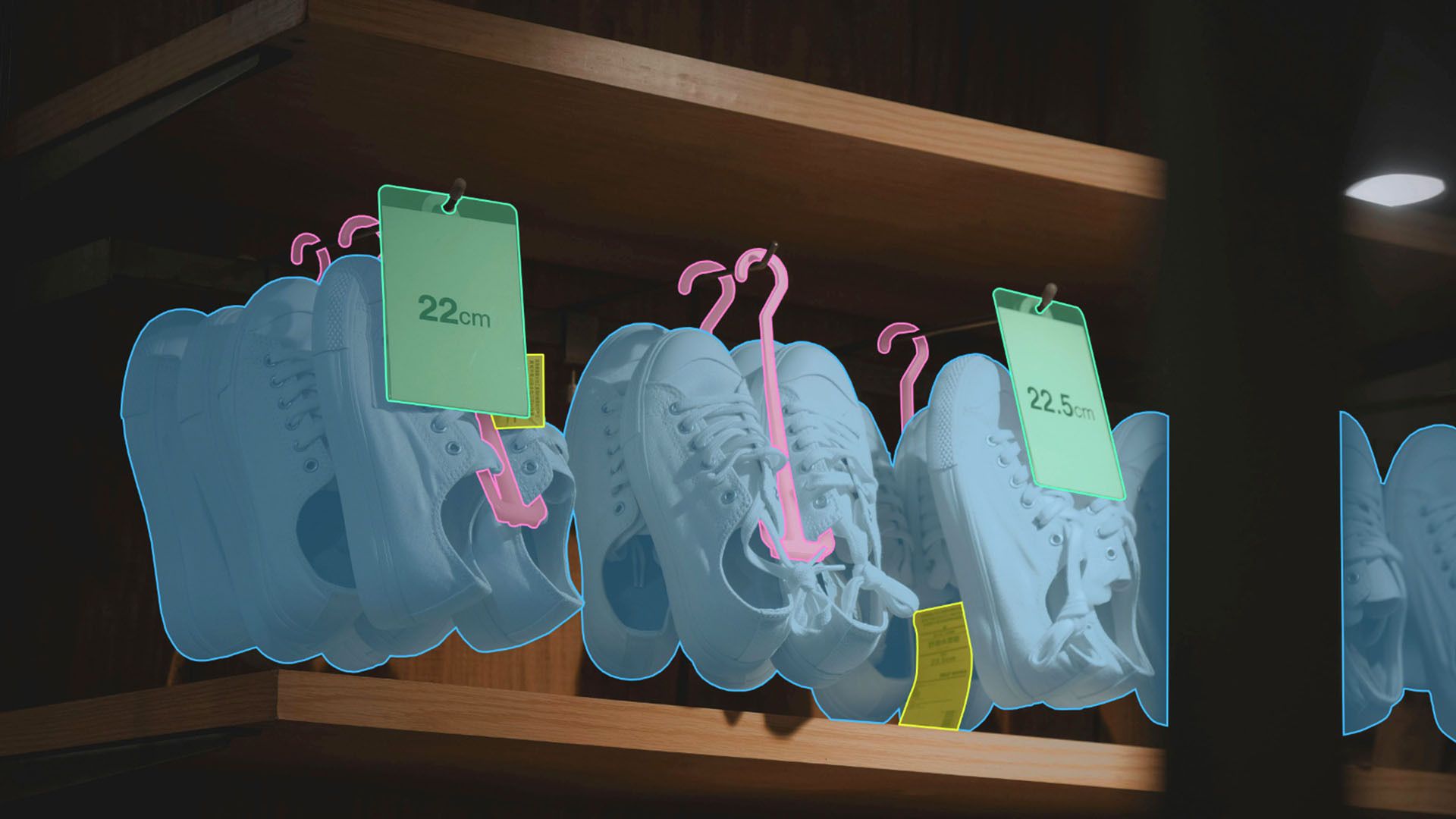
Data annotation for AI in e-commerce plays an important role in today's systems. Picking the best data tagging tools matters a lot for our models - it can lower mistake rates, ensuring top-notch tagged data. Labeling data for personalization empowers customer segmentation with ML, which allows e-commerce platforms to provide unique services to each customer visiting their platform.
Key Takeaways
- Data annotation is crucial for enabling AI-driven customer segmentation in e-commerce
- Quality control in data annotation directly impacts the performance of AI algorithms
- Skilled human-in-the-loop annotators proactively raise edge cases for improved accuracy
- Working with accurate data can 3x time to market for e-commerce ML models

The Role of Data Annotation in AI-Driven Customer Segmentation
In the quick world of online shopping, data annotation is key. It helps AI understand customer groups better by labeling and interpreting huge datasets. This process lets businesses learn lots about what their customers like and how they shop. They use this info to create personalized experiences and boost sales.
Data annotation does more than just make AI better. It also makes online selling smoother and more effective. For example, it makes finding products online easier, boosts how products are sorted, and helps businesses spot trends that can increase sales.
It also helps in a lot of other ways:
- Shows up in more online searches and brings in more visitors by fine-tuning product info
- Makes sorting products faster and better with automatic labels
- Schedules which data to work on first, based on how much it can help reach business goals
- Predicts future buys more accurately and spots chances to sell more to each customer
- Makes it simpler to group customers by their age, shopping records, and interests
- Helps people find items just by using photos
- Gets more sales by studying and perfecting product info plans
By using annotated data, online shops can teach AI to offer spot-on product tips. These tips can make customers happier and boost sales. Good product info can also help a shop rank higher in online searches, giving it an edge over rivals.
Data annotation plays a vital role in training AI models for precise, personalized product recommendations and better customer experience, ultimately driving business growth.
But data annotation isn't just for customers. It can help a business run smoother behind the scenes. It can make sure a store has the right amount of stock, cut down on shipping issues, and lower the number of returned items.
Benefit | Description |
---|---|
Operational Efficiency | Streamlined processes and data-driven decision making |
Supply Chain Optimization | Improved inventory management and reduced shipping errors |
Cost Reduction | Minimized returns, replacements, and additional shipping charges |
Competitive Edge | Increased brand exposure and customer engagement through optimized product data |
As online shopping continues changing, those who use data annotation will do well. This method helps businesses give better service, run smoother, and beat their rivals in the online market.
Key Factors to Consider When Selecting Data Labeling Tools
Starting an AI project means picking the right data labeling tools matters a lot. It can really influence how successful your project is. There are many tools out there, and the AI field is always changing. To choose wisely, think about what your project needs and what you want to achieve.
Support for Required Data Types
Your project's data types are a big deal when choosing a data labeling tool. Projects can need different types of data, like images, video, text, or audio. It's important that your tool can handle the types of data you'll be working with. Keylabs comes in handy here, offering a powerful tool for accurate annotation to enterprises at scale.
Availability of Necessary Annotation Tasks
Being able to do the tasks you need on your data is vital. These could include tasks like image classification or named entity recognition (NER). Pick a tool that can do the specific annotation tasks your project needs. This will make it faster and easier to label your data accurately.
Efficiency of the Annotation Process
How efficient a tool is at labeling data is very important. Look for tools with features that make labeling easier and faster. Here are some things to look for:
- A user-friendly interface can help annotators learn quickly and work faster.
- Collaborative tools make it easy for team members to work together, which speeds up large projects.
- Automated features can help suggest labels or automate tasks, making the process quicker.
- Quality control tools ensure that your labeled data is accurate and consistent.
Choosing a tool that labels data efficiently can save you time and effort. A study by IBM found that many companies saw data complexity as a barrier to AI success. Picking the right labeling tool can help you move past these issues.
The time-to-value and cost-to-value ratios differ from use case to use case. It's essential to evaluate the specific requirements of your project and select a data labeling tool that offers the best balance of efficiency, quality, and cost-effectiveness for your needs.
Consideration | Importance |
---|---|
Support for Required Data Types | Ensures compatibility with your project's specific data needs |
Availability of Necessary Annotation Tasks | Enables efficient labeling of data for your AI application |
Efficiency of the Annotation Process | Streamlines workflow, saves time and resources, and maintains data quality |
Take your time to focus on these important aspects. This approach will help you find the right data labeling tools. Tools that meet your project's needs will help your AI project succeed.
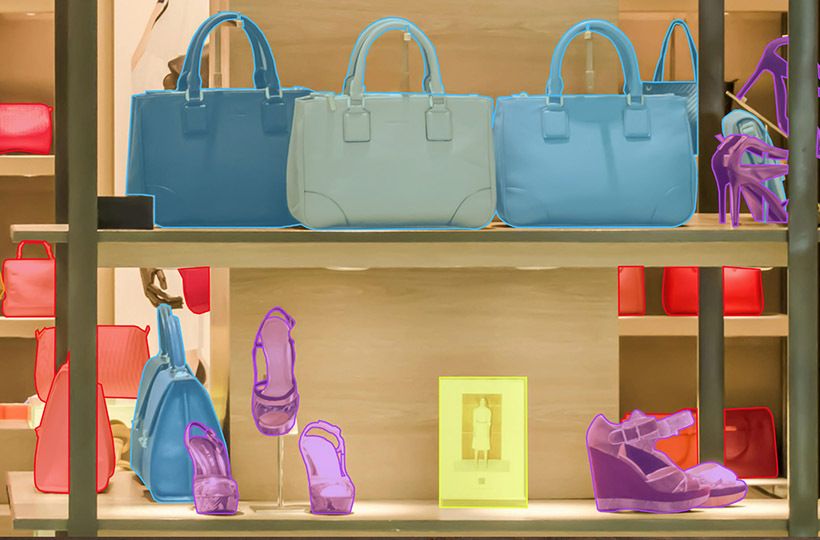
The Importance of Comprehensive Quality Control Mechanisms
Getting the data right every time is vital. That's why we need a wide range of checks during labeling. It includes things like clear rules, spot checks often, fixing errors quickly, and even using machines to look for problems in big sets of data.
This full-check approach cuts mistakes, keeps data together, and makes AI models more trustworthy.
Quality Inspector Roles and Responsibilities
Quality inspectors are key players in data accuracy. They check over the data according to the rules. They fix any mistakes. They help workers get better at their job. Plus, they work with bosses to better the marking process.
They help a lot in running a smooth data project and in building powerful AI models.
Real-time Quality Inspection and Machine Quality Inspection
Two clever methods really help keep a close tab on data quality:
Technique | Description | Benefits |
---|---|---|
Real-time Quality Inspection | Keep an eye on data being labeled to catch errors right away. |
|
Machine Quality Inspection | Let AI do checks on big sets using set rules. This helps find big issues. |
|
Mixing these two methods makes for an awesome system. It ensures AI projects have top-quality data. And that’s essential for their success.
Collaborative Data Annotation for Seamless Teamwork
Data annotation relies heavily on teamwork, making AI projects successful. Teamwork in labeling data speeds up the process and improves quality. It also lets people from different places work together easily.
A good data annotation system should help teams work well. It should split tasks based on what's needed and keep them time tracked. This lets leaders manage resources better and see how everyone is doing.
Dividing work clearly among team members is key in data annotation. By giving each person their role, errors can be reduced. This method makes the annotation process smoother. Training annotators well is crucial for good teamwork. They need the right information and skills to do their job right.
Ongoing training also keeps the team working well together and boosts productivity. At Keymakr, we regularly test our annotators in specific project requirements and create an 'exam' for each project to keep data as accurate as possible.
The Growing Demand for Data Annotation in the NLP Field
Artificial intelligence (AI) is cutting-edge technology that's growing fast. It's driving up the need for well-annotated data, especially in natural language processing (NLP). Annotated data is key for teaching AI models to process human language.
This technology is crucial in creating virtual assistants, chatbots, and other systems. These tools need accurate data to understand us better. As the demand for these tech tools increases, so does the need for annotated data in NLP.
Data annotation in NLP covers a lot of ground, from plain text to audio and more. For regular text, tasks include recognizing named entities, drawing out relations, and classifying texts. Speech tasks involved are recognizing what's being said, separating one voice from another, and even sensing emotions.
Data Type | Annotation Tasks | Applications |
---|---|---|
Text | NER, Relation Extraction, Text Classification, Syntactic Analysis | Sentiment Analysis, Chatbots, Document Classification |
Audio | Speech Recognition, Speaker Separation, Emotion Recognition | Virtual Assistants, Call Center Automation, Voice-based AI |
Image-Text | OCR, Image Captioning, Key Information Extraction | Document Processing, Image Search, Accessibility |
As the NLP data annotation market grows, working with top data annotation services is essential. Quality data helps businesses develop better AI-driven tools. This process improves how users interact with AI, promoting innovation in the industry.
Emerging Trends in Data Annotation Tools
The data annotation market is growing fast. By 2028, it's projected to be worth USD 8.22 billion. This growth is fueled by the sharp rise in unstructured data managed by companies. Thus, there's an increasing need for smart data annotation tools and AI to handle this load efficiently.
The Rise of Intelligent Data Annotation Tools
Advanced AI in data annotation tools is changing how we annotate data. Technologies like few-shot learning and active learning allow for cost cuts and better efficiency. They also let tools adjust based on how annotators work and what they need.
McKinsey says that by 2040, AI might write, speak, and create visuals like the top 25% of people. This shows that AI and data annotation tools are improving very quickly. They're becoming more important in our work.
Leveraging AI Technologies for Enhanced Annotation Efficiency
Integrating AI into data annotation tools is improving how fast and well we annotate data. Its benefits include:
- Automating tasks like tagging images to make annotation faster
- Finding and fixing data issues through analysis
- Helping understand customer behavior for better selling
- Making product searches easier by tagging images
By using AI, businesses can give better custom experiences, boost their online visibility, and grow in the online market.
Data Annotation Tool Feature | Benefit |
---|---|
Few-shot learning | Reduces the amount of labeled data required for training AI models |
Active learning | Selects the most informative data points for annotation, minimizing manual effort |
Incremental learning | Allows AI models to continuously learn and adapt to new data without retraining from scratch |
As data annotation becomes more key in online selling, companies adopting new tools will lead. They'll benefit from AI's power. This will help them stay up in the market.
Ethical Considerations in Data Annotation
AI tech is growing fast in all kinds of businesses. This makes it key to talk about the right way to handle data. Data annotation is the act of tagging and sorting data for AI. It's crucial for making sure AI is fair, private, and safe. It helps AI systems to act the right way and follow ethical rules.
Data privacy and security are big worries in annotating data. The info marked in datasets can be personal or private. So, it needs to stay safe from hacks and misuse. Using strong safety steps like ISO-certified centers and secure platforms is a must. Also, having your team do annotation work can cut the chance of leaks and keep the process in hands you trust.
FAQ
What is the importance of data annotation in AI-driven customer segmentation for e-commerce?
Data annotation is vital for AI to segment e-commerce customers effectively. It offers accurate information for algorithms. This helps in creating personalized experiences that draw in more customers. Quality data annotation is key for AI to work at its best.
What are the key factors to consider when selecting data labeling tools?
Choosing the right data labeling tools means thinking about the data types, like computer vision or NLP. Also important is what annotation tasks they support, such as image classification or NER. Efficiency in the labeling process is a must. Pick a tool that fits the needs of your project and helps you do the work efficiently.
How does quality control impact data annotation?
Quality control is essential in data annotation. It ensures the success of AI algorithms by providing accurate data. A good quality control plan includes checking for correctly annotated data, stopping errors quickly, and checking data in bulk. All of these steps help keep the data accurate.
What are the benefits of collaborative data annotation?
Collaborative data annotation allows for smoother and more flexible work. It helps organize tasks based on the data's needs and keeps track of progress in real-time. Clear roles and oversight, along with tailored training, are vital for a successful project.
How can cost control be achieved in data annotation projects?
Controling annotation costs is crucial for AI companies. The right tool should be cost-effective and not compromise on quality. It should also provide detailed performance data to help manage expenses well.
What is the growing demand for data annotation in the Natural Language Processing (NLP) field?
The need for data annotation in NLP is increasing fast. NLP allows machines to understand and interact with human language. It includes tasks like text, audio, and image-text annotation. This involves efforts such as NER, relation extraction, and speech recognition.
What are the emerging trends in data annotation tools?
Modern data annotation tools incorporate AI to work smarter. Features like few-shot learning and active learning cut down on costs and enhance efficiency. They also help refine data selection and worker roles based on feedback. Such tools can pinpoint data issues and suggest improvements.
What ethical considerations are important in data annotation?
With the rise of AI, ethical data annotation is more important than ever. Issues like bias and privacy highlight the need for fair and transparent practices. Fair pay and diverse teams help ensure trust in AI. Using secure working environments and following ethical guidelines further protect data.
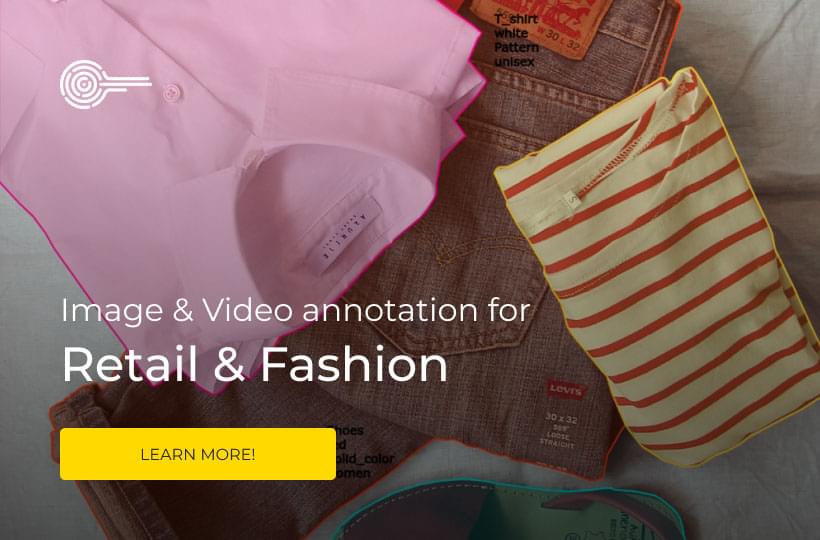