6 steps to writing a POC for your security project
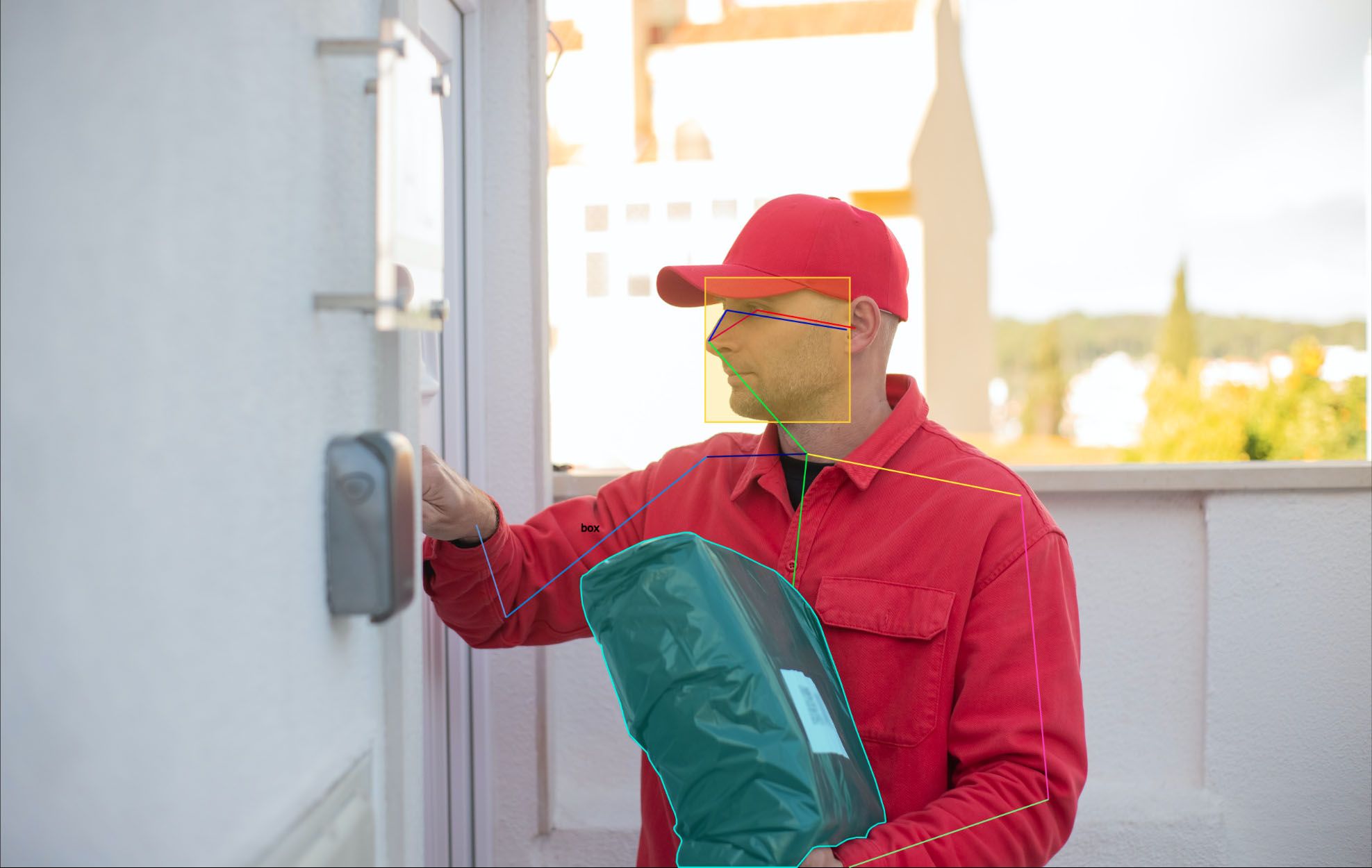
The use of computer vision based AI models for security purposes can be controversial. However, there is little doubt that AI has the capacity to make businesses and public spaces safer. Security cameras are everywhere but it can often be challenging for security staff to monitor them effectively 24/7.
With so much visual information to process it is easy for humans to miss vital signs of crime or potential violence. AI can help fill in this gap by providing constant, smart vigilance of digital security images and video.
However, this sector poses a number of challenges for would-be developers. One of the biggest is securing high quality annotated training data for security AI training. Getting the annotation process right is a vital part of writing a successful security AI proof of concept.
In today's blog we will identify 6 steps that AI teams need to take as part of a profitable security AI project.
Identifying the right AI application for security
There are a range of use cases for AI in the security sector. Identifying the product need is, of course, the first step in the journey of any security project. Examples include:
- Emotion recognition: AI models can be trained to recognise human emotions. Annotated images train these systems to distinguish between normal behavior and potentially problematic emotional expressions. This technology can be useful for security teams, giving them early warning of violent outburst or interactions.
- Facial recognition: Identifying individuals by their facial features is useful for security in sensitive spaces and facilities like airports. AI has the capacity to track thousands of people per second through digital security cameras.
- Dangerous object recognition: AI models can recognise objects like knives and guns. This creates alerts that security staff can respond to quickly.
Defining your annotation methods
Different AI use cases demand different annotation approaches. The following techniques are deployed most often in the world of security AI:
- Key points: This annotation technique locates important points in objects. In training data for facial and emotion recognition AI this means adding a point to the nose, eyes, mouth and ears of an individual in an image.
- Polygon annotation: To accomplish this technique annotators trace around the outline of an object in a digital image. This allows training images to capture the precise shape of important objects like weapons or suitcases.
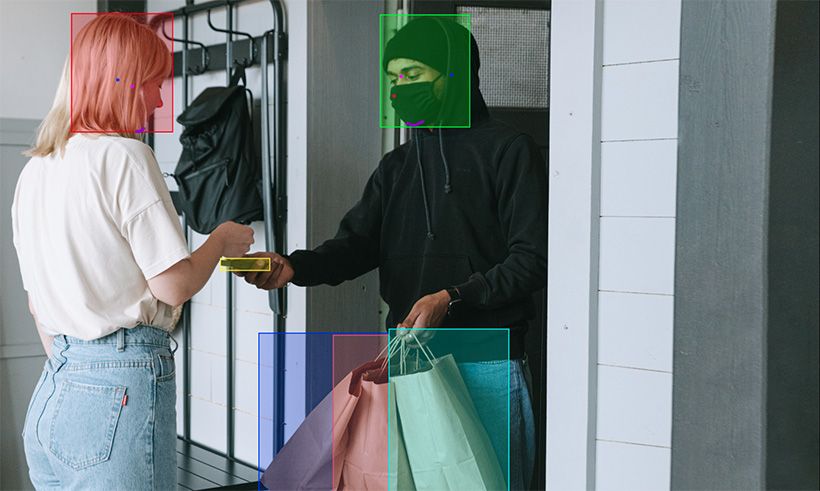
Finding a good annotation workforce
Once security AI teams have defined their data annotation strategy they need to find the right workforce to label their data. Crowdsourced annotation can be cheap but it can also be prone to errors and delays.
Keymakr is an annotation provider with an in-house team of annotators led by experienced managers. Managed annotation teams can maintain high labeling precision and respond quickly to troubleshooting.
Accessing effective annotation tools
The right annotation tool can dramatically streamline image and video labeling. Keymakr’s platform has unique project management features that give developers insights into worker performance.
Annotation verification
Verifying annotations is a crucial part of ensuring effective training datasets for security AI. Keymakr uses three layers of human quality verification in addition to an automated sense check. This process guarantees that labeling mistakes will not impact model performance.
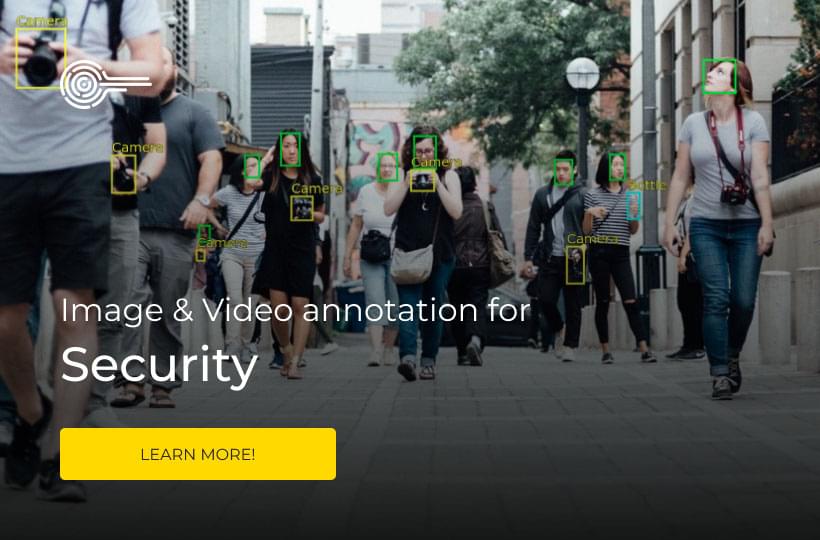
Secure data for security AI training
Images and video for security AI training can be sensitive and require data security protocols. Keymakr follows strict standards, making sure to keep data private and secure by applying measures like encryption, data expiration, and VPNs.
Contact a team member to book your personalized demo today.