3 Reasons why Manual data annotation labor market will survive among automated jungles
As the field of artificial intelligence (AI) continues to grow, the importance of data annotation cannot be understated. Data annotation is the process of labeling data to make it understandable by machines, and it is essential for training AI algorithms. While machine learning algorithms have made great strides in automating data annotation, there are still limitations to their accuracy and reliability. This is where manual data annotation comes in.
Despite the rise of AI, there are still three key reasons why manual data annotation will continue to play a vital role in the labor market. First, human intelligence is essential for complex data annotation tasks that require expert knowledge and judgment. Second, manual data annotation is crucial for quality control and ensuring accuracy in AI models. Finally, the ethics of automated data annotation require human oversight to prevent bias and ensure fairness.
In this article, we will explore these three reasons in more detail and provide case studies of successful applications of manual data annotation in various industries. We will also discuss the skills and training needed for a career in manual data annotation.

The Importance Of Human Intelligence In Data Annotation
Data annotation is a crucial part of artificial intelligence (AI) and machine learning (ML) projects. It involves manually labeling data, which is then fed into AI models for training. Poor data annotation can have severe consequences on the accuracy and effectiveness of AI, which is why companies invest heavily in it.
One reason manual data annotation will survive among AI-related processes relates to quality assurance.When using automated tools without human input, they might classify race incorrectly or miss important features. Humans are needed to assess complex characteristics such as language translation where context determines meaning.Customers demand high-quality work from data annotators, so companies will continue to rely on human annotators for accurate annotations.
Another reason why manual data annotation labor market will always stay relevant is their cost-effectiveness.It’s often thought that automated tools would be cheaper upfront than hiring workers for the job because machines don’t tire out or take breaks.However, it turns out hiring people saves money in the long run since machines have trouble processing certain types of content without guidance from humans. Also, a highly skilled team of trained employees help eliminate mistakes compared to automation farming done overseas.
Data annotators are significant players in generating better forms of AI technology.On a bigger scale, accuracy plays a huge role increasing confidence levels of investors and small businesses alike investing heavily in these technologies.For industries with sensitive applications like healthcare applications requiring accurate analysis , highly-experienced professionals need to generate effective results.Personnel doing trustworthy work gives executives peace-of-mind knowing that customer satisfaction won't falter because algorithms - however advanced- have limitations. While solutions dependent on machines become popular every day,businesses also understand the "artificial intelligence" realm really relies on human intelligence .
The Limitations Of Machine Learning In Data Annotation
Data annotation is an integral part of the machine learning pipeline, allowing algorithms to better understand and interpret information. However, it can also be a time-consuming and resource-intensive process that requires extensive human labor. This presents a significant challenge for organizations looking to implement AI solutions in their operations.
One reason why manual data annotation will likely survive among AI labor markets is that the human eye is still much more adept at certain tasks, such as identifying new objects in images. While there are efforts to automate these processes through AI tools, they have not yet reached a level of precision and accuracy that can match human capabilities.
Another reason why manual data annotation may remain relevant is related to potential biases in machine learning. The source of data used for training an algorithm can affect its performance and lead to unforeseen results if not properly accounted for. Human annotators offer a level of bias detection and mitigation that cannot currently be matched by automated processes alone.
Finally, it's worth noting that while there are challenges associated with manual data annotation, there is also a rapidly growing market for data annotation services. This suggests that demand for skilled annotators will remain high as organizations continue to invest in AI solutions.
While technological advancements continue to improve the efficiency of automated data annotation processes, it's clear that skilled human labor will remain critical in ensuring the quality and accuracy of these systems. As such, investing in building up this talent remains key to continued success in the field of machine learning and AI.
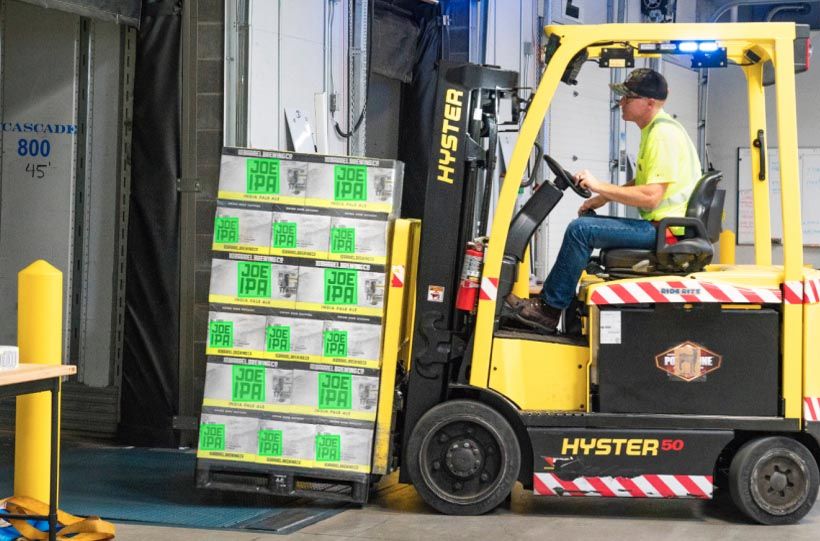
The Benefits Of Manual Data Annotation For Quality Control
Manual data annotation has been proved to be highly effective in quality control compared to automated tools. There are several key benefits of manual data annotation for quality control.
Firstly, human annotators are more efficient and accurate, resulting in fewer mistakes and lower costs. Leveraging human expertise ensures greater accuracy in labeling data as it is done through a trained eye that undergoes rigorous testing, unlike an automated system. Additionally, manual labeling tools can better capture edge cases that may be missed by automated labeling tools.
Secondly, quality control is crucial in ensuring accurate results. Quality testing minimizes errors and delivers consistent noise-free data so that the final model is more relevant for businesses or projects.
Lastly, In-house labeling maximizes security as there's minimal risk of sensitive data getting leaked externally since privacy policies are upheld within the company. This reduces the likelihood of regulatory sanctions associated with failure to adhere to regulatory compliance measures imposed on sensitive business or government-related content.
While crowdsourced data labeling offers cost advantages, quality control cannot always be guaranteed as unqualified individuals may participate. Companies need to weigh their options carefully before outsourcing such tasks depending on various factors like project requirements and budget restrictions while considering leveraging human labor market vendors with experience in technical expertise needed when manually annotating documents like expert linguists or medical professionals provided by companies like LionBridge AI services.
Businesses across different verticals collaborating with machine learning algorithms can benefit significantly from manual data processing techniques for document annotation since it entails developing better models leading to improved ROI at reduced cost if correctly implemented for quality control purposes both internally using their workforce or external service providers while keeping closely monitoring progress concerning accuracy levels continuously updated towards industry-wide benchmarks being set up over time depending on task complexity level required.
The Role Of Human Expertise In Complex Data Annotation Tasks
Human expertise is essential in complex data annotation tasks, particularly in Natural Language Processing (NLP) and Computer Vision (CV) domains. The use of human-in-the-loop methods is critical for fast and precise data annotation, enabling annotators to adapt quickly to new tasks. Humans are valuable in selecting and gathering the right typology of data and labeling it appropriately for AI projects.
The complexity of data-to-text tasks makes defining accuracy difficult, which means deviations from the truth are inevitable. This highlights the importance of Subject Matter Expertise (SME) when preparing data for models to train on. The involvement of industry experts early on can ensure high-quality datasets with accurate annotations that aid machine learning algorithms to achieve high precision rates.
Expert input can also help companies avoid a common challenge presented by using automated annotation tools called the 'dirty label' problem – where pre-existing biases in machine learning datasets are carried over into the final models. Human annotators provide some degree of subjectivity that helps machines overcome bias while still maintaining a reasonable level of accuracy during the training process.
Despite technological advances that promise large-scale automation, there will always be a need for manual labor in complex data annotation tasks like NLP and CV applications. As such, businesses need qualified people from different backgrounds – including linguists, expert domain knowledge personnel - involved at every stage: from dataset creation through documentation all the way up until testing model performance. As market demand grows worldwide – reflected by projections estimating an impressive 26% CAGR from 2023-2030 - more households should consider investing time into learning crucial skills that would make them viable candidates within this field's industry standards.
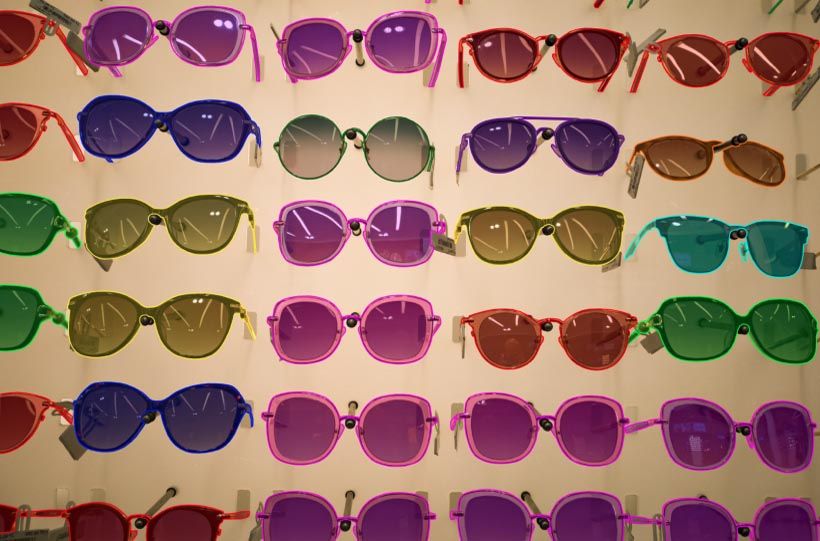
The Future Of Manual Data Annotation In The Age Of AI
Manual data labeling has been and will continue to be the leader in data annotation due to its accuracy and reliability. Despite the growth of AI, manual annotation remains vital as it offers a human touch, which AI cannot provide. The industry is expected to grow exponentially in the coming years. As demand increases for more efficient and accurate data labeling, services are becoming more sophisticated.
However, certain tasks can disturb annotators during the annotation process and compromise its efficiency. For example, fatigue or burnout might occur if annotators work long hours with a lot of repetitive tasks. It’s important that companies address these concerns by providing support or breaks to ensure an accurate labeling process continues.
As machine learning technology continues advancing, manual data annotation becomes increasingly crucial because AI needs properly labeled training data to learn from historical patterns effectively. Thus, this service will remain essential as machine learning applications keep on rising across different industries like robotics and self-driving cars.
We cannot overlook the importance of manual data annotation in today's world of fast-paced technological advancements. Through continued improvements in annotator support systems combined with enhanced sophistication within the industry itself like gamification using crowdsourcing for human annotators will increase productivity while maintaining high levels of quality control necessary for reliable results so no doubt existing labor market domination will push forward unabatedly toward future progressions tout suite!
The Ethics Of Automated Data Annotation And The Need For Human Oversight
Automated data annotation has been gaining popularity due to its efficiency and cost-effectiveness. However, relying solely on automation can create issues related to bias and errors. In order to prevent these problems, human oversight is necessary to validate results and identify anomalies.
One of the major concerns associated with automated data annotation is potential biases. Automated metadata annotation depends heavily on the training dataset or rules available for a particular domain. If there are any biases in this dataset, it can be reflected in the annotations leading to biased algorithms that learn from these annotations.
Another issue with automation is that it may not always be feasible or scalable for large or complex datasets. Fully automating labeling tasks can be time-consuming, labor-intensive, and costly which may make it difficult for some businesses.
Human-annotated data is still considered one of the most reliable methods of data annotation since it provides higher accuracy than automated approaches can provide alone. Although manual annotation requires investment in labor costs, employing manual annotators utilizing existing crowd-sourcing platforms also provide an opportunity for more significant representation from different perspectives improving ethical considerations.
Automated metadata annotation offers a cost-effective alternative but may cause unexpected issues like biases during process execution due to lack of reliable training sets resulting in outputs requiring added human oversight. As such investing in both automated labels approaches plus human validation procedures will ensure better outcomes by decreasing chances of errors created by either solely utilizing either approach alone thus making human supervision critical especially when working with sensitive information that require accurate and responsible gathering processes such as medical information collections augmented lending risk evaluation dataset especially calibrated taking into account unbiased gender/race factors etcetera called Fairness-Aware Machine Learning techniques .
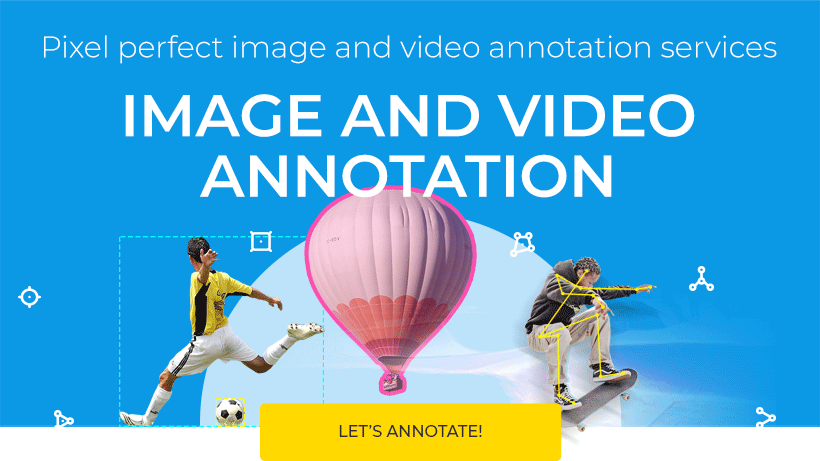
Case Studies: Successful Applications Of Manual Data Annotation In Various Industries
Manual data annotation services are necessary for various industries that require accurate and reliable machine learning and AI applications. Human supervision (data validation) in the annotation process is critical as it adds value to the algorithm, making it perform better. A quality dataset reduces cost and time, thus allowing businesses to make informed decisions quickly.
One industry where manual data labeling services have thrived is healthcare. Healthcare organizations need an efficient way of analyzing medical records with accurate diagnosis codes that can be implemented in clinical decision support systems for future treatments. Hiring teams of medical coders to manually annotate medical records ensures that treatment errors reduce remarkably.
Another industry where manual data annotation is vital is banking and finance operations. Banks require high levels of precision when conducting risk assessments and detecting fraudulent behavior among their customers' transactions or credit reports. The introduction of regulations such as Know Your Customer (KYC), Anit-Money Laundering (AML) increased demand for tailored solutions from providers who offer high-quality annotating services.
Finally, e-commerce companies also benefit significantly from accurate product categorization using visual search technologies enhanced by optimal feature selection through manual data tagging by professional annotators who provide relevant metadata. This service is particularly useful for image analytics firms that leverage on object identification algorithms to understand consumer's engagement on set products accurately.
The labor market will continue surviving because these three industries continuously require well-labeled datasets to improve their customer experience resulting in businesses making informed decisions faster while hiring teams committed to delivering quality annotations within specific deadlines, which requires consistency, accuracy, focus on detail, excellent communication skills and timely delivery at all times.
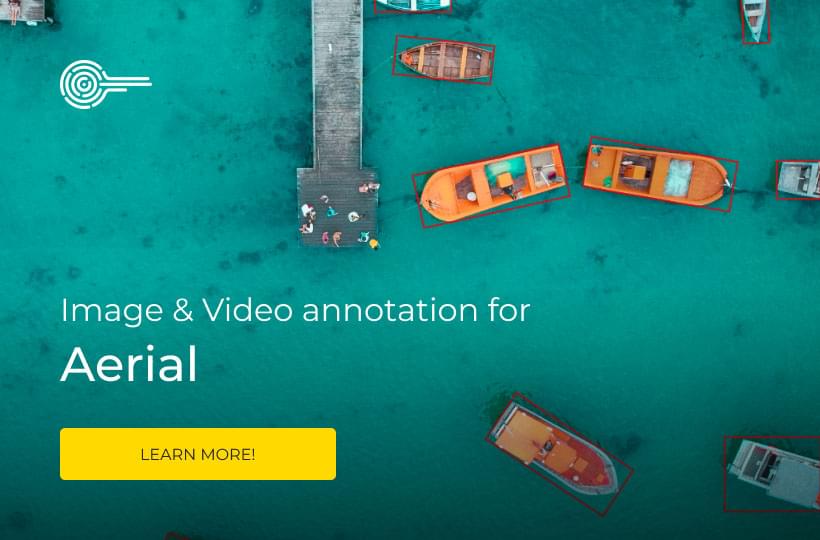