Data Creation vs. Open Source Data
Open source data sets are good resources that contain pre-existing, publicly available data. Data creation is new data that is generated or collected. After it is created, new data may be made open source. Although it may be protected data, like patient data, or it may be otherwise restricted. Because open source data is, well, open source, it is pretty much free for everyone to use. There is no reason not to use open source data. You can include that free and open source in a larger custom data set.
On the other hand, data creation from things like new pictures and videos may be better for your specific project. It may also be better suited. That is because open source data was often not originally made for machine learning. Whereas, say, video data collection for machine learning has your goal of using data to train an AI in mind from the beginning. Of course, you can always count on us for your data creation needs.
You may also not find the kind of data you need for your project in an open source data set. For example, people can be pretty protective of things like the precision agriculture data collection they have done. Such data may provide a competitive advantage or tip off competitors to some problems that a farm is experiencing. Believe it or not, agricultural data of certain kinds may even be of interest to national security.
While it would be very nice if all data could be free and open source, there are good reasons it is not. That means you will probably need data collection and labeling services like those we provide for your project. Usually, you want to include the most relevant data you can in your data set to avoid bias anyway. Using data collection and annotation that is unique and tailored to your project's needs can help you create a better product.
Open source data is sometimes the product of what is known as data exhaust. Data exhaust can still be useful but may be incomplete or have other issues. Data exhaust is a kind of by-product. Open source data may also come from many disparate sources. It can also be misinterpreted. It can also be old and of lower quality.
Another important consideration is that open source data is still also copyright data. That means that you have to comply with the open source license or licenses that the data is under. You can not use all open source data for commercial use. Certainly, training an AI for a product you intend to sell is a commercial use. There are many different kinds of open source licenses that are all based on copyright law. You may need a lawyer or an entire legal team to help you understand the various licenses and comply with them.
Data created for you to use usually does not have any license or copyright to worry about. That is great because an AI project is already very complicated, with a lot of moving parts that can be difficult to manage. It can be pretty much just your company's data if you want, so you control it. You can make it open source and generally available or keep it proprietary.
The Pros and Cons of Open Source Data
- One pro is increased explainability and transparency, which builds trust. A real con is that depending on the data, there may be concerns about privacy and consent.
- Open source data can provide opportunities for community engagement and contributions.
- A pro and con in one are that open source data is highly accessible.
- Open source data can improve efficiency and reduce costs. That is always a big plus.
- Another privacy concern of open source data is the mosaic effect. Anonymized data may not stay anonymous when enough different bits and pieces appear in different public data sets.
- Open source licensing may prevent commercial use and has various rules to follow. That can be a problem.
Data Creation For Your Next Innovation
Data creation can ensure that you have sovereignty over the data set you use to train your AI. That means you can use that data for your commercial products. You can also better maintain privacy, which is often a real concern. That includes privacy for people whose data may be included in some form and privacy for your company and project. In cybersecurity, we learn that privacy is an integral part of security.
Another nice thing about data creation is that you can set all parameters to suit your needs. Such data can be validated and also increase explainability. With good data creation, you have more control over your data and the entire process of creating your data set, labeling, and training your model. It is also possible to assure that data is high quality using video data collection services or image data collection services such as ours.
Using the best practices of data creation also makes the data set better for making predictions. That is because more information can be collected to put the data into context. It is important to
remember that you often don't have to choose between open source data or data creation. Rather you can incorporate open source data using our data collection and labeling services to benefit your project.
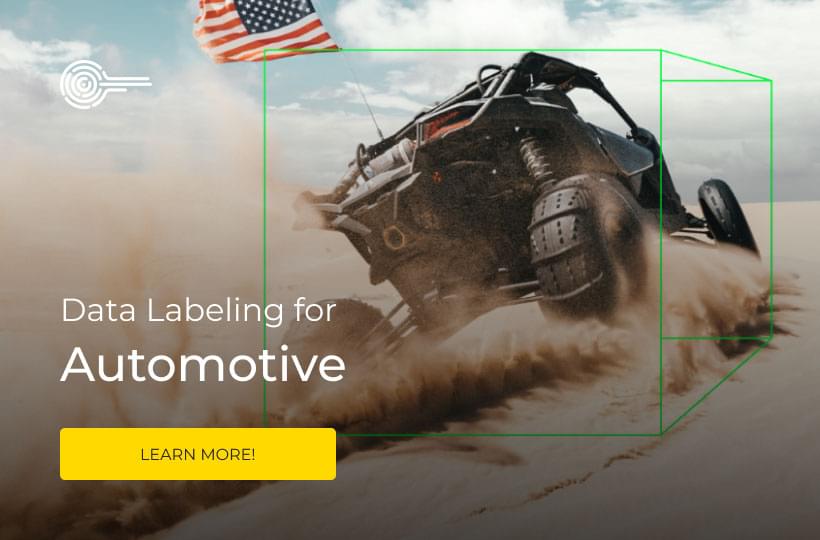