Cuboid Annotation Improves Automotive AI Performance
Automotive AI models have the potential to drastically improve safety on our roads. Automated vehicles should be able to navigate traffic reliably and avoid accidents at a higher rate than human drivers. For AI models in this fast growing sector reliability is absolutely essential, failures could lead to high profile accidents that damage public trust in future generations of self-driving vehicles.
Therefore it is vital that computer vision AI models are trained with highly accurate annotated training images and video. This training data must also reflect the complexity and spatial dimensions of the real, three dimensional world. As a result data annotation providers, like Keymakr, are now offering cuboid annotation as part of their suite of labeling techniques.
In today’s blog we will talk about cuboid annotation and how it can produce more functional training datasets. We will also look at how cuboid annotation can work in partnership with professional annotation services to allow automotive AI projects to flourish.
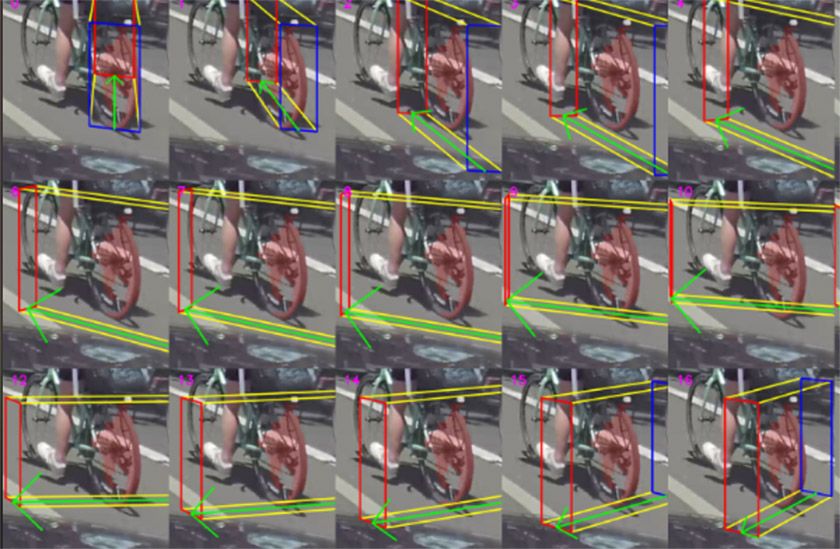
AI automotive applications
Cuboid annotation creates data that replicates the movements and interactions present in complex traffic environments. The following capabilities, enabled by data annotation, are essential for safe automated travel:
- Object detection: Object detection allows autonomous vehicles to identify and respond to specific objects. During video and image annotation human annotators locate and then assign labels to cars, trucks, buses, etc. Through exposure to training data annotated in this way computer vision models are able to recognize things in the world around them and respond accordingly.
- Environmental perception: Annotators use semantic segmentation techniques to create training data that labels every pixel in an image or video frame. This vital context allows AIs to understand their surroundings in more detail.
- Understanding signage: Autonomous vehicles can automatically detect road signs and respond to them accordingly. Annotation services can enable this use case with careful video labeling.
- Lane recognition: In order to be deployed safely on public roads automated vehicle models must be able to recognize lane markings and stay within them. Annotation helps define the parallel shapes of lanes, allowing autonomous vehicles to stay within safe limits.
Cuboid annotation
Training data for autonomous vehicles is made up of two dimensional images and frames of three dimensional spaces. Most of the time we use bounding boxes to locate objects and people when annotating for AI training and we can use bounding boxes to add dimensions to these objects. Take an image of a car.
Annotators will place a bounding box around the front of the vehicle and then one at the end of the vehicle, by joining the corners of these boxes together we can produce a shape that reflects approximate dimensions. This kind of annotation allows AI models to understand where they are in three dimensional space. This added level of detail will help to improve the safety and performance of these models.
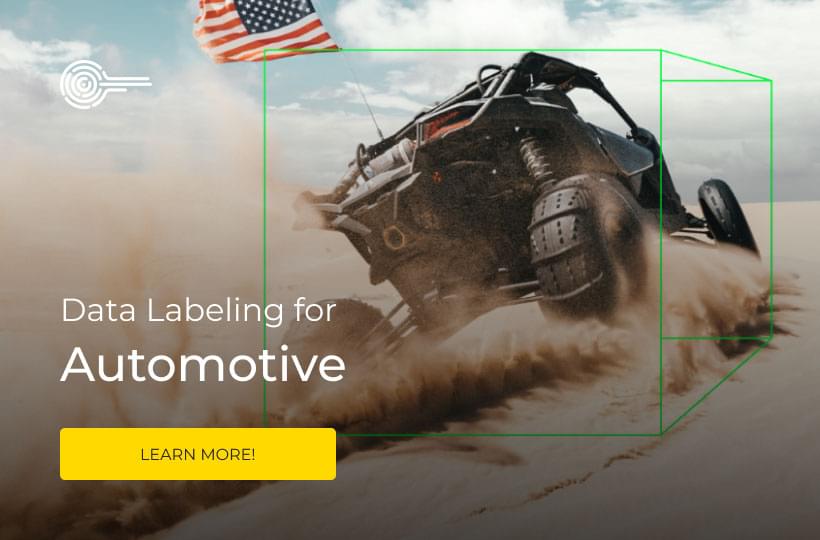
Cuboid annotation services
It is essential that AI developers have access to high quality annotation services if they are to make the most of cuboid annotation. Keymakr is an annotation provider with an in-house annotation team led by experienced managers.
Keymakr’s cost effective services are accurate and scalable, and give developers unique insights into the performance of their annotation workforce.
Contact a team member to book your personalized demo today.