Choosing the Right Data Annotation Platform
Data annotation is a crucial step in training machine learning algorithms. It involves tagging, transcribing, or labeling specific features in datasets to train the ML system. To achieve accurate and reliable results, it is important to choose the right data annotation platform. This article will highlight the key factors to consider when selecting an annotation tool, including managing datasets, annotation techniques, workforce management, and security.
Key Takeaways:
- Choosing the right data annotation platform is essential for successful machine learning data annotation.
- Consider factors such as managing datasets, annotation techniques, workforce management, and security when selecting an annotation tool.
- Verify that the tool has secure file access and restricted viewing rights to ensure data protection.
- Select a tool that is versatile, flexible, and scalable to accommodate future requirements.
- Engage/train your workforce to effectively utilize the annotation tool and maximize its benefits for your AI projects.

Managing Datasets
When it comes to choosing a data annotation tool, managing datasets is a crucial consideration. The tool you select should have the capability to handle the volume of data and the variety of file types that need to be annotated. This ensures that your data annotation process can be executed efficiently and effectively, leading to accurate machine learning outcomes.
An important feature to look for in a data annotation tool is its ability to search, filter, clone, sort, and merge datasets. These functionalities allow you to organize and manipulate your data easily, making it more manageable and accessible for the annotation process. By having these tools at your disposal, you can streamline your workflow and save valuable time and effort.
Another key factor to consider is the compatibility of the data annotation tool with your file storage systems. Seamless integration between the tool and your existing storage systems is crucial for efficient data management. It enables smooth access to datasets and ensures that any changes made during the annotation process are properly saved and stored.
Choosing a data annotation tool with robust managing capabilities not only simplifies the process of organizing and manipulating datasets but also improves the overall efficiency and accuracy of the annotation process.
To illustrate the importance of managing datasets effectively, here is an example:
Challenge | Solution |
---|---|
You have a large dataset with thousands of images in various file formats. | Select a data annotation tool that can handle large volumes of data and support multiple file types, ensuring seamless annotation. |
Your dataset needs to be filtered, sorted, and merged before annotation to eliminate duplicates and ensure consistency. | Choose a tool that offers search, filter, sort, and merge functionalities for datasets, allowing you to organize and manipulate your data efficiently. |
Your dataset is stored in a cloud-based file storage system. | Ensure that the data annotation tool is compatible with your cloud storage system, enabling easy access and synchronization of datasets. |
By effectively managing your datasets through the right data annotation tool, you can streamline the annotation process, enhance productivity, and achieve accurate machine learning outcomes.
Image: Managing Datasets - Ensure efficient data management through effective dataset organization and manipulation.
Annotation Techniques
In the field of data annotation, the annotation techniques used can vary depending on the specific requirements of a project. Data annotation tools play a vital role in facilitating the application of these techniques. Different tools offer different capabilities, ranging from specialized annotation tools to general annotation tools.
Specialized annotation tools are designed to annotate specific types of labeling, providing a focused approach for specific use cases. These tools excel in accuracy and efficiency when working with specific annotation tasks. For example, image annotation tools are specifically designed to annotate and label objects, regions, or attributes within an image. Similarly, video annotation tools are tailored for annotating videos, while text annotation tools are used for labeling text data, such as sentiment analysis or named entity recognition.
On the other hand, general annotation tools offer more flexibility by providing a wide range of annotation techniques for various types of data. These tools can accommodate multiple use cases and annotation tasks. They may include features such as bounding box annotation, polygon annotation, semantic segmentation, and more. General annotation tools are ideal for organizations that work with diverse datasets and require a versatile solution to handle different annotation requirements.
Annotation Techniques Examples:
- Image Annotation: Annotating objects, regions, or attributes within an image.
- Video Annotation: Annotating videos for object tracking, action recognition, or scene understanding.
- Text Annotation: Labeling text data for sentiment analysis, named entity recognition, or text classification.
- Audio Annotation: Annotating audio files for speech recognition or sound event detection.
Choosing the Right Annotation Tool:
When selecting an annotation tool, it is crucial to consider the current and future needs of your organization. Evaluate the complexity of your annotation tasks and determine whether a specialized or a general tool would be a better fit. It's also important to consider the scalability and flexibility of the tool to adapt to evolving annotation requirements.
Below is an example of a comparison between specialized and general annotation tools:
Specialized Annotation Tools | General Annotation Tools |
---|---|
High accuracy and efficiency for specific annotation tasks | Flexibility to handle multiple annotation techniques |
Limited applicability to specific use cases | Ability to handle diverse datasets and annotation requirements |
Streamlined workflows for specific annotation tasks | Versatility for accommodating different use cases |
By considering the benefits and trade-offs of specialized and general annotation tools, you can make an informed decision that aligns with your organization's annotation needs, ensuring optimal results for your machine learning and AI projects.
annotation tools
Workforce Management
Data annotation tools play a crucial role in training machine learning algorithms, but they require human intervention for handling exceptions and ensuring high-quality annotations. Effective workforce management is essential to optimize productivity and maximize the potential of annotators. When choosing a data annotation tool, it's important to look for features that facilitate workforce management and productivity optimization.
Evaluate annotation tools that offer productivity tracking functionalities, allowing you to monitor the performance and progress of annotators. This feature enables you to identify bottlenecks, allocate resources efficiently, and streamline the annotation process. With real-time productivity insights, you can identify areas where additional training or support might be needed to enhance the performance of your annotators.
Task assignment is another critical aspect of workforce management. Find a data annotation tool that allows you to assign tasks to annotators based on their skill level and expertise. This ensures that the most suitable annotators are assigned to each annotation task, leading to better accuracy and efficiency. By effectively managing task assignments, you can distribute workload evenly among your annotators and prevent burnout or fatigue.
An important decision to make is whether to hire annotators from external vendors or train your existing workforce to use the annotation tool efficiently. Hiring external annotators can provide specialized expertise and scalability, but it may come at a higher cost. On the other hand, training your existing workforce allows you to leverage their domain knowledge and familiarity with your organization's processes.
"Effective workforce management is crucial to optimize productivity and maximize the potential of annotators."
Benefits of Workforce Management Features |
---|
1. Improved productivity and efficiency |
2. Real-time performance tracking |
3. Effective task assignments based on annotator skills |
4. Resource allocation and workload distribution |
5. Cost optimization through strategic decision-making |
With effective workforce management, you can optimize the annotation process, minimize errors, and improve the overall quality of your machine learning data. By considering productivity tracking, task assignments, and the right workforce strategy, you can maximize the potential of your annotators and achieve optimal results.
Security
Security is a crucial aspect when selecting data annotation tools. Organizations must prioritize data protection by ensuring that the tool they choose provides robust security features. Two primary security concerns to consider are secure file access and restricted viewing rights.
Secure file access ensures that only authorized individuals can access and manipulate the annotated data. This feature prevents unauthorized access and potential data breaches. The tool should have built-in mechanisms to authenticate users and provide secure access to files.
Restricted viewing rights further enhance data protection by limiting access to sensitive information. Organizations can assign specific viewing privileges to different user roles or teams, ensuring that only authorized personnel can view confidential or sensitive data.
"Data protection is critical in data annotation, where privacy and confidentiality are of utmost importance."
When selecting a data annotation tool, it is essential for organizations to pay attention to the specific security requirements of their industry and internal policies. Regulatory compliance and standards such as GDPR (General Data Protection Regulation) and HIPAA (Health Insurance Portability and Accountability Act) must be adhered to.
Data Annotation Tool Comparison: Security Features
Data Annotation Tool | Secure File Access | Restricted Viewing Rights |
---|---|---|
Annotate Pro | ✅ | ✅ |
LabelMaster | ✅ | ✅ |
AnnoLab | ✅ | ✅ |
LabelGenius | ✅ | ✅ |
AnnoShield | ✅ | ✅ |
Note: The data provided in the table above is for illustrative purposes only and does not reflect the complete list of data annotation tools available in the market.
By choosing a data annotation tool with robust security features, organizations can ensure the protection of their sensitive data and maintain compliance with industry regulations.
Types of Data Annotation Tools
When it comes to data annotation, there are various types of tools available in the market to cater to different requirements. These tools play a crucial role in annotating specific types of data, such as text, images, or videos. They also offer consolidated platforms that can handle different types of data, providing a comprehensive solution for annotation needs. It's important to consider the features and functionalities of each tool to make an informed decision.
Text Annotation Tools
Text annotation tools are specifically designed to annotate textual data. They enable the identification of entities, sentiments, or any other relevant information within the text. These tools assist in labeling and categorizing textual data for various applications, such as natural language processing, sentiment analysis, and text classification.
Image Annotation Tools
Image annotation tools are essential for annotating images with labels, bounding boxes, polygons, or keypoints. These tools are widely used in computer vision tasks, including object detection, image segmentation, and facial recognition. Image annotation tools allow for the creation of high-quality labeled datasets to train machine learning models effectively.
Video Annotation Tools
Video annotation tools are specifically designed to annotate videos by labeling objects, tracking their movements, or identifying specific actions within the video frames. These tools assist in tasks such as object tracking, activity recognition, and video summarization. Video annotation tools are crucial for training models that analyze and understand videos.
AI Development Process
The AI development process heavily relies on data annotation tools to generate accurately labeled datasets. These tools aid in preparing training data for machine learning models, enabling AI systems to learn and make predictions effectively. By utilizing data annotation tools, organizations can streamline the AI development process and improve the performance of their models.
"Data annotation tools play a vital role in the AI development process, enabling the efficient labeling and categorization of different types of data. From text annotation to image and video annotation, these tools provide the necessary support for training machine learning models."
Future Requirements
When selecting a data annotation tool, it is essential to take into account the future needs of your business. You should opt for a tool that can adapt to the evolving requirements of your organization, providing versatility, flexibility, and scalability. Additionally, it is crucial to ensure that the chosen tool is compatible with your storage systems, whether they are cloud-based, network-based, or utilizing local storage.
Investing in a data annotation tool that can meet future requirements is crucial to avoid the need for costly tool migrations or replacements down the line. By carefully considering scalability and compatibility with storage systems, you can future-proof your data annotation processes and seamlessly integrate the tool with your existing infrastructure.
For organizations that anticipate substantial growth or evolving data annotation needs, a scalable tool is highly advantageous. Scalability allows you to handle increasing volumes of data and annotations without compromising efficiency. By choosing a tool that can accommodate your future requirements, you can ensure that your data annotation efforts remain seamless and reliable throughout your organization's growth.
Compatibility with your storage systems is equally important. Whether you store your data in the cloud, on-premises, or through a network-based solution, the data annotation tool should seamlessly integrate with your existing infrastructure. This compatibility ensures efficient data access, smooth workflows, and effective collaboration among team members.
Overall, by considering the future requirements of your business, including scalability and compatibility with storage systems, you can select a data annotation tool that aligns with your long-term goals. This careful consideration will set the foundation for successful data annotation processes and enable your organization to effectively leverage the value of annotated data for machine learning and AI development.
Popular Data Annotation Tools
When it comes to data annotation, there are several popular tools available in the market. Organizations operating at scale often prefer commercially-viable data annotation tools, which they can customize to fulfill their specific business requirements. On the other hand, open-source data annotation tools allow for modification of the source code, providing the flexibility to customize the tool according to specific needs. To make an informed decision, it is essential to consider the pros and cons of each type of tool.
Commercial Data Annotation Tools
Commercial data annotation tools are designed to meet the needs of organizations operating at scale. These tools offer a range of advanced features and functionalities, making them ideal for complex annotation projects. The advantages of using commercial tools include:
- Customizability: Commercial tools can be customized to align with specific business requirements, offering a tailored experience.
- Advanced Features: These tools often provide a wide range of annotation techniques and support various data types such as images, text, videos, and more.
- Technical Support: Users of commercial tools typically have access to dedicated technical support, ensuring a seamless annotation process.
Open-Source Data Annotation Tools
Open-source data annotation tools provide a high level of flexibility as they allow users to modify the source code of the tool. This customization capability makes open-source tools popular among organizations that have specific annotation requirements. The advantages of using open-source tools include:
- Flexibility: Users have the freedom to modify the tool according to their specific needs, enabling customization at a granular level.
- Community Support: Open-source tools often have an active community that provides support and shares knowledge, offering assistance in troubleshooting and optimization.
- Cost-Effective Solution: Open-source tools are typically free to use, making them an attractive option for organizations with budget constraints.
When choosing between commercial and open-source data annotation tools, it is important to consider factors such as budget, project complexity, customization requirements, and available technical resources. It is also worth exploring the user reviews and ratings of different tools to gain insights into their performance and usability.
Commercial Data Annotation Tools | Open-Source Data Annotation Tools |
---|---|
Tool A | Tool X |
Tool B | Tool Y |
Tool C | Tool Z |
Importance of Data Scientists
To achieve successful data annotation, it's crucial to have not just the right tools, but also skilled laborers such as data scientists. Data scientists play a critical role in supporting the annotation process and ensuring high-quality outcomes for your AI projects. Their expertise in analyzing and interpreting data enables them to make informed decisions during the annotation process.
Skilled data scientists possess the knowledge and experience to identify patterns, outliers, and nuances in the data that may impact the annotation strategy. They can also provide valuable insights and recommendations on selecting the most appropriate annotation techniques and tools for specific use cases.
Furthermore, data scientists can effectively leverage the annotation tool's features and functionalities to optimize the annotation workflow and improve overall efficiency. Their ability to navigate complex datasets and understand the underlying AI models helps in achieving accurate and reliable annotations.
"Data scientists act as the bridge between the data annotation tool and the desired AI outcomes. Their expertise in data analysis and understanding of machine learning principles contribute significantly to the success of the annotation process."
To harness the full potential of data annotation tools, it is recommended to engage and train your workforce in the efficient utilization of these tools. Provide necessary training sessions to enhance their understanding of the annotation process and the specific functionalities of the chosen tool.
By empowering your team with the knowledge and skills to effectively use the annotation tool, you can ensure smoother collaboration and maximize the benefits of data annotation in your AI projects.
Benefits of Data Scientists in the Annotation Process
Data scientists bring several advantages to the annotation process:
- Expertise in data analysis and interpretation to make informed annotation decisions
- Ability to identify patterns, outliers, and nuances in the data for accurate annotations
- Understanding of machine learning principles to align annotations with AI objectives
- Optimization of annotation workflows for improved efficiency and productivity
- Recommendations on the use of annotation techniques and tools for specific use cases
Having data scientists as part of your annotation team ensures a comprehensive and well-informed approach to data labeling, leading to high-quality training datasets for your machine learning models.
Conclusion
Choosing the right data annotation platform is paramount for the success of your AI projects. By carefully considering factors such as managing datasets, annotation techniques, workforce management, security, and future requirements, you can make an informed decision.
There are various data annotation platforms available, including both commercial and open-source options. Commercial tools offer customization and scalability, making them ideal for large-scale operations. On the other hand, open-source tools provide flexibility for modifying the source code to suit specific needs.
However, having the right tool is only part of the equation. It is equally important to have a skilled workforce in place to effectively utilize the annotation platform. Data scientists and knowledgeable laborers can ensure the seamless application of the tool, resulting in high-quality machine learning data annotation.
In conclusion, selecting the appropriate data annotation platform, evaluating the available options, and employing a competent workforce are the key steps for achieving effective data annotation, streamlining the AI development process, and driving successful outcomes for your business.
FAQ
What is data annotation?
Data annotation involves tagging, transcribing, or labeling specific features in datasets to train machine learning systems.
How do I choose the right data annotation platform?
When selecting a data annotation platform, consider factors such as managing datasets, annotation techniques, workforce management, and security.
What should I consider when choosing a data annotation tool?
Consider the tool's capability to support the volume and file types of data, as well as its search, filter, clone, sort, and merge functionalities. Integration with your file storage systems is also important.
What are the different annotation techniques used by data annotation tools?
Data annotation tools can perform image annotation, video annotation, text annotation, and audio annotation. Choose a tool that meets your organization's specific needs.
How can I optimize workforce management in data annotation?
Look for annotation tools that offer workforce management features like productivity tracking and efficient task assignments. You can hire annotators from external vendors or train your existing workforce.
What security features should I consider in a data annotation tool?
Verify that the tool has secure file access and restricted viewing rights to protect your data. Choose a tool that aligns with your organization's security requirements.
What types of data annotation tools are available in the market?
Data annotation tools can be specialized for annotating specific types of data (text, images, videos) or offer a consolidated platform for various data types. Consider the features and enrichment capabilities of each tool.
How should I consider future requirements when choosing a data annotation tool?
Select a versatile, flexible, and scalable tool that aligns with your organization's evolving needs. Compatibility with your storage systems is crucial, whether they are cloud-based, network-based, or local.
What are some popular data annotation tools in the market?
There are commercially-viable tools that can be customized for specific business requirements and open-source tools that allow for modification of the source code. Weigh the pros and cons before making a decision.
How important is a skilled workforce in the data annotation process?
Having skilled data scientists and laborers is essential for successful annotation. Engage and train your workforce to effectively utilize the annotation tool and ensure high-quality outcomes for your AI projects.
Why is choosing the right data annotation platform important?
The right data annotation platform is crucial for the success of your AI projects. Consider factors such as managing datasets, annotation techniques, workforce management, security, and future requirements to make an informed choice.
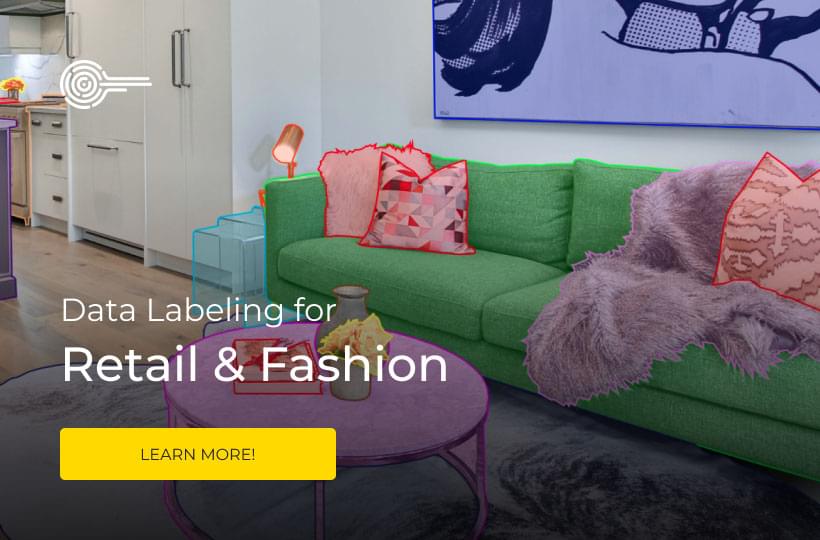